Open Access
ARTICLE
Efficient Classification of Remote Sensing Images Using Two Convolution Channels and SVM
1 ENSA, Abdelmalek Essaadi University, Tetouan 93002, Morocco
2 FS, Abdelmalek Essaadi University, Tetouan 93002, Morocco
3 Department of Electrical Engineering, Faculty of Engineering, Menoufia University, Shebin El-Kom 32511, Egypt
4 Department of Computer Engineering, College of Computers and Information Technology, Taif University, P.O. Box 11099, Taif 21944, Saudi Arabia
5 ENSIAS, Mohammed V University in Rabat 10000, Morocco
6 Department of Information Technology, College of Computers and Information Technology, Taif University, P.O. Box 11099, Taif 21944, Saudi Arabia
* Corresponding Author: Mohammed Baz. Email:
Computers, Materials & Continua 2022, 72(1), 739-753. https://doi.org/10.32604/cmc.2022.022457
Received 08 August 2021; Accepted 01 December 2021; Issue published 24 February 2022
Abstract
Remote sensing image processing engaged researchers’ attentiveness in recent years, especially classification. The main problem in classification is the ratio of the correct predictions after training. Feature extraction is the foremost important step to build high-performance image classifiers. The convolution neural networks can extract images’ features that significantly improve the image classifiers’ accuracy. This paper proposes two efficient approaches for remote sensing images classification that utilizes the concatenation of two convolution channels’ outputs as a features extraction using two classic convolution models; these convolution models are the ResNet 50 and the DenseNet 169. These elicited features have been used by the fully connected neural network classifier and support vector machine classifier as input features. The results of the proposed methods are compared with other antecedent approaches in the same experimental environments. Evaluation is based on learning curves plotted during the training of the proposed classifier that is based on a fully connected neural network and measuring the overall accuracy for the both proposed classifiers. The proposed classifiers are used with their trained weights to predict a big remote sensing scene's classes for a developed test. Experimental results ensure that, compared with the other traditional classifiers, the proposed classifiers are further accurate.Keywords
Cite This Article
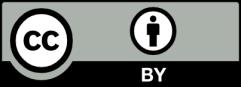
This work is licensed under a Creative Commons Attribution 4.0 International License , which permits unrestricted use, distribution, and reproduction in any medium, provided the original work is properly cited.