Open Access
ARTICLE
An Optimal Deep Learning for Cooperative Intelligent Transportation System
1 Department of Computer Science and Engineering, Periyar Maniammai Institute of Science & Technology, Thanjavur, 613403, India
2 Department of Computer Science and Engineering, KG Reddy College of Engineering and Technology, Telangana, 500075, India
3 Department of Computer Science and Engineering, Vignan’s Institute of Information Technology (Autonomous), Visakhapatnam, 530049, India
4 Department of Computer Science and Engineering, Kalasalingam Academy of Research and Education, Krishnankoil, 626128, India
5 Department of Computer Engineering, College of Engineering Pune, Pune, Maharashtra, 411005, India
6 Department of Entrepreneurship and Logistics, Plekhanov Russian University of Economics, Moscow, 117997, Russia
7 Department of Logistics, State University of Management, Moscow, 109542, Russia
* Corresponding Author: Irina V. Pustokhina. Email:
Computers, Materials & Continua 2022, 72(1), 19-35. https://doi.org/10.32604/cmc.2022.020244
Received 15 May 2021; Accepted 16 June 2021; Issue published 24 February 2022
Abstract
Cooperative Intelligent Transport System (C-ITS) plays a vital role in the future road traffic management system. A vital element of C-ITS comprises vehicles, road side units, and traffic command centers, which produce a massive quantity of data comprising both mobility and service-related data. For the extraction of meaningful and related details out of the generated data, data science acts as an essential part of the upcoming C-ITS applications. At the same time, prediction of short-term traffic flow is highly essential to manage the traffic accurately. Due to the rapid increase in the amount of traffic data, deep learning (DL) models are widely employed, which uses a non-parametric approach for dealing with traffic flow forecasting. This paper focuses on the design of intelligent deep learning based short-term traffic flow prediction (IDL-STFLP) model for C-ITS that assists the people in various ways, namely optimization of signal timing by traffic signal controllers, travelers being able to adapt and alter their routes, and so on. The presented IDL-STFLP model operates on two main stages namely vehicle counting and traffic flow prediction. The IDL-STFLP model employs the Fully Convolutional Redundant Counting (FCRC) based vehicle count process. In addition, deep belief network (DBN) model is applied for the prediction of short-term traffic flow. To further improve the performance of the DBN in traffic flow prediction, it will be optimized by Quantum-behaved bat algorithm (QBA) which optimizes the tunable parameters of DBN. Experimental results based on benchmark dataset show that the presented method can count vehicles and predict traffic flow in real-time with a maximum performance under dissimilar environmental situations.Keywords
Cite This Article
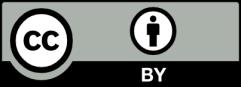
This work is licensed under a Creative Commons Attribution 4.0 International License , which permits unrestricted use, distribution, and reproduction in any medium, provided the original work is properly cited.