Open Access
ARTICLE
Optimized Deep Learning Model for Colorectal Cancer Detection and Classification Model
1 Information Technology Department, Faculty of Computing and Information Technology, King Abdulaziz University, Jeddah, 21589, Saudi Arabia
2 Center of Artificial Intelligence for Precision Medicines, King Abdulaziz University, Jeddah, 21589, Saudi Arabia
3 Department of Mathematics, Faculty of Science, Al-Azhar University, Naser City, 11884, Cairo, Egypt
4 Department of Pharmacy Practice, Faculty of Pharmacy, King Abdulaziz University, Jeddah, 21589, Saudi Arabia
5 Information Systems Department, Faculty of Computing and Information Technology, King Abdulaziz University, Jeddah, 21589, Saudi Arabia
6 Electrical and Computer Engineering Department, Faculty of Engineering, King Abdulaziz University, Jeddah, 21589, Saudi Arabia
7 Computer Science Department, Faculty of Computers and Information, Luxor University, 85951, Egypt
8 Mathematics Department, Faculty of Science, South Valley University, Qena, 83523, Egypt
9 Department of Mathematics, Faculty of Science, Taif University, Taif, 21944, Saudi Arabia
* Corresponding Author: Mahmoud Ragab. Email:
Computers, Materials & Continua 2022, 71(3), 5751-5764. https://doi.org/10.32604/cmc.2022.024658
Received 26 October 2021; Accepted 08 December 2021; Issue published 14 January 2022
Abstract
The recent developments in biological and information technologies have resulted in the generation of massive quantities of data it speeds up the process of knowledge discovery from biological systems. Due to the advancements of medical imaging in healthcare decision making, significant attention has been paid by the computer vision and deep learning (DL) models. At the same time, the detection and classification of colorectal cancer (CC) become essential to reduce the severity of the disease at an earlier stage. The existing methods are commonly based on the combination of textual features to examine the classifier results or machine learning (ML) to recognize the existence of diseases. In this aspect, this study focuses on the design of intelligent DL based CC detection and classification (IDL-CCDC) model for bioinformatics applications. The proposed IDL-CCDC technique aims to detect and classify different classes of CC. In addition, the IDL-CCDC technique involves fuzzy filtering technique for noise removal process. Moreover, water wave optimization (WWO) based EfficientNet model is employed for feature extraction process. Furthermore, chaotic glowworm swarm optimization (CGSO) based variational auto encoder (VAE) is applied for the classification of CC into benign or malignant. The design of WWO and CGSO algorithms helps to increase the overall classification accuracy. The performance validation of the IDL-CCDC technique takes place using benchmark Warwick-QU dataset and the results portrayed the supremacy of the IDL-CCDC technique over the recent approaches with the maximum accuracy of 0.969.Keywords
Cite This Article
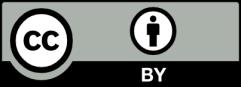
This work is licensed under a Creative Commons Attribution 4.0 International License , which permits unrestricted use, distribution, and reproduction in any medium, provided the original work is properly cited.