Open Access
ARTICLE
Deep Reinforcement Learning Enabled Smart City Recycling Waste Object Classification
1 Department of Natural and Applied Sciences, College of Community-Aflaj, Prince Sattam bin Abdulaziz University, Saudi Arabia
2 Department of Information Systems-Girls Section, King Khalid University, Mahayil, 62529, Saudi Arabia
3 Department of Computer Science, King Khalid University, Muhayel Aseer, Saudi Arabia
4 Faculty of Computer and IT, Sana'a University, Sana'a, Yemen
5 Department of Information Systems, King Khalid University, Muhayel Aseer, 62529, Saudi Arabia
6 Department of Computer and Self Development, Preparatory Year Deanship, Prince Sattam bin Abdulaziz University, AlKharj, Saudi Arabia
7 Department of Information Systems, College of Computer and Information Sciences, Princess Nourah Bint Abdulrahman University, Saudi Arabia
8 Department of Mathematics, Faculty of Science, Cairo University, Giza, 12613, Egypt
* Corresponding Author: Manar Ahmed Hamza. Email:
Computers, Materials & Continua 2022, 71(3), 5699-5715. https://doi.org/10.32604/cmc.2022.024431
Received 17 October 2021; Accepted 29 November 2021; Issue published 14 January 2022
Abstract
The Smart City concept revolves around gathering real time data from citizen, personal vehicle, public transports, building, and other urban infrastructures like power grid and waste disposal system. The understandings obtained from the data can assist municipal authorities handle assets and services effectually. At the same time, the massive increase in environmental pollution and degradation leads to ecological imbalance is a hot research topic. Besides, the progressive development of smart cities over the globe requires the design of intelligent waste management systems to properly categorize the waste depending upon the nature of biodegradability. Few of the commonly available wastes are paper, paper boxes, food, glass, etc. In order to classify the waste objects, computer vision based solutions are cost effective to separate out the waste from the huge dump of garbage and trash. Due to the recent developments of deep learning (DL) and deep reinforcement learning (DRL), waste object classification becomes possible by the identification and detection of wastes. In this aspect, this paper designs an intelligence DRL based recycling waste object detection and classification (IDRL-RWODC) model for smart cities. The goal of the IDRL-RWODC technique is to detect and classify waste objects using the DL and DRL techniques. The IDRL-RWODC technique encompasses a two-stage process namely Mask Regional Convolutional Neural Network (Mask RCNN) based object detection and DRL based object classification. In addition, DenseNet model is applied as a baseline model for the Mask RCNN model, and a deep Q-learning network (DQLN) is employed as a classifier.Moreover, a dragonfly algorithm (DFA) based hyperparameter optimizer is derived for improving the efficiency of the DenseNet model. In order to ensure the enhanced waste classification performance of the IDRL-RWODC technique, a series of simulations take place on benchmark dataset and the experimental results pointed out the better performance over the recent techniques with maximal accuracy of 0.993.Keywords
Cite This Article
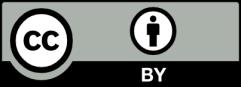
This work is licensed under a Creative Commons Attribution 4.0 International License , which permits unrestricted use, distribution, and reproduction in any medium, provided the original work is properly cited.