Open Access
ARTICLE
Machine Learning Based Analysis of Real-Time Geographical of RS Spatio-Temporal Data
Department of Disaster Management, Al-Balqa Applied University, Prince Al-Hussien Bin Abdullah II Academy for Civil Protection, Jordan
* Corresponding Author: Rami Sameer Ahmad Al Kloub. Email:
Computers, Materials & Continua 2022, 71(3), 5151-5165. https://doi.org/10.32604/cmc.2022.024309
Received 13 October 2021; Accepted 15 November 2021; Issue published 14 January 2022
Abstract
Flood disasters can be reliably monitored using remote sensing photos with great spatiotemporal resolution. However, satellite revisit periods and extreme weather limit the use of high spatial resolution images. As a result, this research provides a method for combining Landsat and MODIS pictures to produce high spatiotemporal imagery for flood disaster monitoring. Using the spatial and temporal adaptive reflectance fusion model (STARFM), the spatial and temporal reflectance unmixing model (STRUM), and three prominent algorithms of flexible spatiotemporal data fusion (FSDAF), Landsat fusion images are created by fusing MODIS and Landsat images. Then, to extract flood information, utilize a support vector machine (SVM) to classify the fusion images. Assess the accuracy of your work. Experimental results suggest that the three spatio-temporal fusion algorithms may be used to effectively monitor floods, with FSDAF's fusion results outperforming STARFM and STRUM in both study areas. The overall flood classification accuracy of the three STARFM, STRUM, and FSDAF algorithms in the Gwydir research region is 0.89, 0.90, and 0.91, respectively, with Kappa coefficients of 0.63, 0.64, and 0.67. The flood classification accuracy of the three fusion algorithms in the New Orleans research region is 0.90, 0.89, and 0.91, with Kappa values of 0.77, 0.76, and 0.81, respectively. The spatio-temporal fusion technique can be used to successfully monitor floods, according to this study.Keywords
Cite This Article
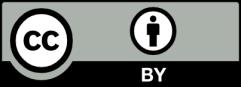
This work is licensed under a Creative Commons Attribution 4.0 International License , which permits unrestricted use, distribution, and reproduction in any medium, provided the original work is properly cited.