Open Access
ARTICLE
Human Faces Detection and Tracking for Crowd Management in Hajj and Umrah
1 Department of Information Systems and Technology, College of Computer Science and Engineering, University of Jeddah, Jeddah, Saudi Arabia
2 Electrical Engineering Department, College of Engineering, Northern Border University, Arar, Saudi Arabia
3 Laboratory of Electronics and Microelectronics (LR99ES30), Faculty of Sciences of Monastir, University of Monastir, Tunisia
4 College of Computer Sciences, King Khalid University, Abha, Saudi Arabia
5 CES Laboratory, ENIS, University of Sfax, Tunisia
* Corresponding Author: Yahia Said. Email:
Computers, Materials & Continua 2022, 71(3), 6275-6291. https://doi.org/10.32604/cmc.2022.024272
Received 11 October 2021; Accepted 14 December 2021; Issue published 14 January 2022
Abstract
Hajj and Umrah are two main religious duties for Muslims. To help faithfuls to perform their religious duties comfortably in overcrowded areas, a crowd management system is a must to control the entering and exiting for each place. Since the number of people is very high, an intelligent crowd management system can be developed to reduce human effort and accelerate the management process. In this work, we propose a crowd management process based on detecting, tracking, and counting human faces using Artificial Intelligence techniques. Human detection and counting will be performed to calculate the number of existing visitors and face detection and tracking will be used to identify all the humans for security purposes. The proposed crowd management system is composed form three main parts which are: (1) detecting human faces, (2) assigning each detected face with a numerical identifier, (3) storing the identity of each face in a database for further identification and tracking. The main contribution of this work focuses on the detection and tracking model which is based on an improved object detection model. The improved Yolo v4 was used for face detection and tracking. It has been very effective in detecting small objects in high-resolution images. The novelty contained in this method was the integration of the adaptive attention mechanism to improve the performance of the model for the desired task. Channel wise attention mechanism was applied to the output layers while both channel wise and spatial attention was integrated in the building blocks. The main idea from the adaptive attention mechanisms is to make the model focus more on the target and ignore false positive proposals. We demonstrated the efficiency of the proposed method through expensive experimentation on a publicly available dataset. The wider faces dataset was used for the train and the evaluation of the proposed detection and tracking model. The proposed model has achieved good results with 91.2% of mAP and a processing speed of 18 FPS on the Nvidia GTX 960 GPU.Keywords
Cite This Article
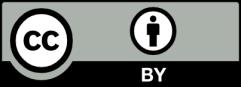