Open Access
ARTICLE
Accurate Location Estimation of Smart Dusts Using Machine Learning
1 Institute of Applied Data Analytics (IADA), Universiti Brunei Darussalam (UBD), Gadong, BE1410, Brunei Darussalam
2 School of Digital Sciences (SDS), Universiti Brunei Darussalam (UBD), Gadong, BE1410, Brunei Darussalam
* Corresponding Author: Shariq Bashir. Email:
(This article belongs to the Special Issue: Emergent Computer-Based Methods and Internet of Things Technologies for Physical Therapy, Dentistry, Medicine, and Engineering)
Computers, Materials & Continua 2022, 71(3), 6165-6181. https://doi.org/10.32604/cmc.2022.024269
Received 11 October 2021; Accepted 30 November 2021; Issue published 14 January 2022
Abstract
Traditional wireless sensor networks (WSNs) are not suitable for rough terrains that are difficult or impossible to access by humans. Smart dust is a technology that works with the combination of many tiny sensors which is highly useful for obtaining remote sensing information from rough terrains. The tiny sensors are sprinkled in large numbers on rough terrains using airborne distribution through drones or aircraft without manually setting their locations. Although it is clear that a number of remote sensing applications can benefit from this technology, but the small size of smart dust fundamentally restricts the integration of advanced hardware on tiny sensors. This raises many challenges including how to estimate the location of events sensed by the smart dusts. Existing solutions on estimating the location of events sensed by the smart dusts are not suitable for monitoring rough terrains as these solutions depend on relay sensors and laser patterns which have their own limitations in terms of power constraint and uneven surfaces. The study proposes a novel machine learning based localization algorithm for estimating the location of events. The approach utilizes timestamps (time of arrival) of sensed events received at base stations by assembling them into a multi-dimensional vector and input to a machine learning classifier for estimating the location. Due to the unavailability of real smart dusts, we built a simulator for analysing the accuracy of the proposed approach for monitoring forest fire. The experiments on the simulator show reasonable accuracy of the approach.Keywords
Cite This Article
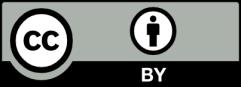
This work is licensed under a Creative Commons Attribution 4.0 International License , which permits unrestricted use, distribution, and reproduction in any medium, provided the original work is properly cited.