Open Access
ARTICLE
A Hybrid Meta-Classifier of Fuzzy Clustering and Logistic Regression for Diabetes Prediction
1 Department of Information Technology, Faculty of Computing and Information Technology in Rabigh, King Abdulaziz University, Jeddah, Saudi Arabia
* Corresponding Author: Altyeb Altaher Taha. Email:
Computers, Materials & Continua 2022, 71(3), 6089-6105. https://doi.org/10.32604/cmc.2022.023848
Received 23 September 2021; Accepted 02 November 2021; Issue published 14 January 2022
Abstract
Diabetes is a chronic health condition that impairs the body's ability to convert food to energy, recognized by persistently high levels of blood glucose. Undiagnosed diabetes can cause many complications, including retinopathy, nephropathy, neuropathy, and other vascular disorders. Machine learning methods can be very useful for disease identification, prediction, and treatment. This paper proposes a new ensemble learning approach for type 2 diabetes prediction based on a hybrid meta-classifier of fuzzy clustering and logistic regression. The proposed approach consists of two levels. First, a base-learner comprising six machine learning algorithms is utilized for predicting diabetes. Second, a hybrid meta-learner that combines fuzzy clustering and logistic regression is employed to appropriately integrate predictions from the base-learners and provide an accurate prediction of diabetes. The hybrid meta-learner employs the Fuzzy C-means Clustering (FCM) algorithm to generate highly significant clusters of predictions from base-learners. The predictions of base-learners and their fuzzy clusters are then employed as inputs to the Logistic Regression (LR) algorithm, which generates the final diabetes prediction result. Experiments were conducted using two publicly available datasets, the Pima Indians Diabetes Database (PIDD) and the Schorling Diabetes Dataset (SDD) to demonstrate the efficacy of the proposed method for predicting diabetes. When compared with other models, the proposed approach outperformed them and obtained the highest prediction accuracies of 99.00% and 95.20% using the PIDD and SDD datasets, respectively.Keywords
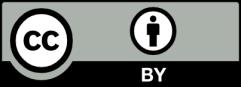