Open Access
ARTICLE
Enhancing Task Assignment in Crowdsensing Systems Based on Sensing Intervals and Location
1 Faculty of Computers and Information, Mansoura University, Mansoura, 35516, Egypt
2 Faculty of Computers and Artificial Intelligence, Benha University, Banha, 13518, Egypt
3 Faculty of Computer Science and Engineering, Galala University, Suez, 435611, Egypt
4 College of Computer and Information Systems, Umm Al-Qura University, Mecca, 715, Saudi Arabia
* Corresponding Author: Louai Alarabi. Email:
Computers, Materials & Continua 2022, 71(3), 5619-5638. https://doi.org/10.32604/cmc.2022.023716
Received 18 September 2021; Accepted 23 November 2021; Issue published 14 January 2022
Abstract
The popularity of mobile devices with sensors is captivating the attention of researchers to modern techniques, such as the internet of things (IoT) and mobile crowdsensing (MCS). The core concept behind MCS is to use the power of mobile sensors to accomplish a difficult task collaboratively, with each mobile user completing much simpler micro-tasks. This paper discusses the task assignment problem in mobile crowdsensing, which is dependent on sensing time and path planning with the constraints of participant travel distance budgets and sensing time intervals. The goal is to minimize aggregate sensing time for mobile users, which reduces energy consumption to encourage more participants to engage in sensing activities and maximize total task quality. This paper introduces a two-phase task assignment framework called location time-based algorithm (LTBA). LTBA is a framework that enhances task assignment in MCS, whereas assigning tasks requires overlapping time intervals between tasks and mobile users’ tasks and the location of tasks and mobile users’ paths. The process of assigning the nearest task to the mobile user's current path depends on the ant colony optimization algorithm (ACO) and Euclidean distance. LTBA combines two algorithms: (1) greedy online allocation algorithm and (2) bio-inspired travel-distance-balance-based algorithm (B-DBA). The greedy algorithm was sensing time interval-based and worked on reducing the overall sensing time of the mobile user. B-DBA was location-based and worked on maximizing total task quality. The results demonstrate that the average task quality is 0.8158, 0.7093, and 0.7733 for LTBA, B-DBA, and greedy, respectively. The sensing time was reduced to 644, 1782, and 685 time units for LTBA, B-DBA, and greedy, respectively. Combining the algorithms improves task assignment in MCS for both total task quality and sensing time. The results demonstrate that combining the two algorithms in LTBA is the best performance for total task quality and total sensing time, and the greedy algorithm follows it then B-DBA.Keywords
Cite This Article
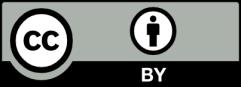
This work is licensed under a Creative Commons Attribution 4.0 International License , which permits unrestricted use, distribution, and reproduction in any medium, provided the original work is properly cited.