Open Access
ARTICLE
Transfer Learning-based Computer-aided Diagnosis System for Predicting Grades of Diabetic Retinopathy
1 College of Computer and Information Sciences, Imam Mohammad Ibn Saud Islamic University (IMSIU), Riyadh, 11432, Saudi Arabia
2 Department of Electrical Engineering, Benha Faculty of Engineering, Benha University, Qalubia, Benha, 13518, Egypt
* Corresponding Author: Qaisar Abbas. Email:
(This article belongs to the Special Issue: Innovative Technology For Machine Intelligence)
Computers, Materials & Continua 2022, 71(3), 4573-4590. https://doi.org/10.32604/cmc.2022.023670
Received 16 September 2021; Accepted 25 October 2021; Issue published 14 January 2022
Abstract
Diabetic retinopathy (DR) diagnosis through digital fundus images requires clinical experts to recognize the presence and importance of many intricate features. This task is very difficult for ophthalmologists and time-consuming. Therefore, many computer-aided diagnosis (CAD) systems were developed to automate this screening process of DR. In this paper, a CAD-DR system is proposed based on preprocessing and a pre-train transfer learning-based convolutional neural network (PCNN) to recognize the five stages of DR through retinal fundus images. To develop this CAD-DR system, a preprocessing step is performed in a perceptual-oriented color space to enhance the DR-related lesions and then a standard pre-train PCNN model is improved to get high classification results. The architecture of the PCNN model is based on three main phases. Firstly, the training process of the proposed PCNN is accomplished by using the expected gradient length (EGL) to decrease the image labeling efforts during the training of the CNN model. Secondly, the most informative patches and images were automatically selected using a few pieces of training labeled samples. Thirdly, the PCNN method generated useful masks for prognostication and identified regions of interest. Fourthly, the DR-related lesions involved in the classification task such as micro-aneurysms, hemorrhages, and exudates were detected and then used for recognition of DR. The PCNN model is pre-trained using a high-end graphical processor unit (GPU) on the publicly available Kaggle benchmark. The obtained results demonstrate that the CAD-DR system outperforms compared to other state-of-the-art in terms of sensitivity (SE), specificity (SP), and accuracy (ACC). On the test set of 30,000 images, the CAD-DR system achieved an average SE of 93.20%, SP of 96.10%, and ACC of 98%. This result indicates that the proposed CAD-DR system is appropriate for the screening of the severity-level of DR.Keywords
Cite This Article
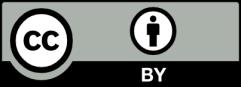