Open Access
ARTICLE
SVM and KNN Based CNN Architectures for Plant Classification
1 Lovely Professional University, Jalandhar, 144005, India
2 Department of Computer Science and Engineering, Chandigarh University, Mohali, India
3 School of Computer Science and Engineering, Tailor's University, Subang Jaya, 47500, Malaysia
4 Department of Computer Science, College of Computers and Information Technology, Taif University, Taif, 21944, Saudi Arabia
* Corresponding Author: Kavita. Email:
Computers, Materials & Continua 2022, 71(3), 4257-4274. https://doi.org/10.32604/cmc.2022.023414
Received 07 September 2021; Accepted 19 October 2021; Issue published 14 January 2022
Abstract
Automatic plant classification through plant leaf is a classical problem in Computer Vision. Plants classification is challenging due to the introduction of new species with a similar pattern and look-a-like. Many efforts are made to automate plant classification using plant leaf, plant flower, bark, or stem. After much effort, it has been proven that leaf is the most reliable source for plant classification. But it is challenging to identify a plant with the help of leaf structure because plant leaf shows similarity in morphological variations, like sizes, textures, shapes, and venation. Therefore, it is required to normalize all plant leaves into the same size to get better performance. Convolutional Neural Networks (CNN) provides a fair amount of accuracy when leaves are classified using this approach. But the performance can be improved by classifying using the traditional approach after applying CNN. In this paper, two approaches, namely CNN + Support Vector Machine (SVM) and CNN + K-Nearest Neighbors (kNN) used on 3 datasets, namely LeafSnap dataset, Flavia Dataset, and MalayaKew Dataset. The datasets are augmented to take care all the possibilities. The assessments and correlations of the predetermined feature extractor models are given. CNN + kNN managed to reach maximum accuracy of 99.5%, 97.4%, and 80.04%, respectively, in the three datasets.Keywords
Cite This Article
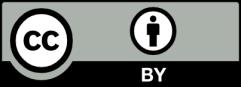