Open Access
ARTICLE
Rainfall Forecasting Using Machine Learning Algorithms for Localized Events
1 Centre for Disaster Mitigation and Management, Vellore Institute of Technology, Vellore, 632014, India
2 School of Computer Science and Engineering, Vellore Institute of Technology, Vellore, 632014, India
3 Department of Computer Science and Information Engineering, National Yunlin University of Science and Technology, Yunlin, 64002, Taiwan
4 Service Systems Technology Center, Industrial Technology Research Institute, Hsinchu, Taiwan
5 School of Information Technology and Engineering, Vellore Institute of Technology, Vellore, 632014, India
6 Geophysical Institute of Vladikavkaz Scientific Centre, Russian Academy of Sciences (RAS), Vladikavkaz, Russian Federation
* Corresponding Author: Chuan-Yu Chang. Email:
Computers, Materials & Continua 2022, 71(3), 6333-6350. https://doi.org/10.32604/cmc.2022.023254
Received 01 September 2021; Accepted 16 December 2021; Issue published 14 January 2022
Abstract
A substantial amount of the Indian economy depends solely on agriculture. Rainfall, on the other hand, plays a significant role in agriculture–while an adequate amount of rainfall can be considered as a blessing, if the amount is inordinate or scant, it can ruin the entire hard work of the farmers. In this work, the rainfall dataset of the Vellore region, of Tamil Nadu, India, in the years 2021 and 2022 is forecasted using several machine learning algorithms. Feature engineering has been performed in this work in order to generate new features that remove all sorts of autocorrelation present in the data. On removal of autocorrelation, the data could be used for performing operations on the time-series data, which otherwise could only be performed on any other regular regression data. The work uses forecasting techniques like the AutoRegessive Integrated Moving Average (ARIMA) and exponential smoothening, and then the time-series data is further worked on using Long Short Term Memory (LSTM). Later, regression techniques are used by manipulating the dataset. The work is benchmarked with several evaluation metrics on a test dataset, where XGBoost Regression technique outperformed the test. The uniqueness of this work is that it forecasts the daily rainfall for the year 2021 and 2022 in Vellore region. This work can be extended in the future to predict rainfall over a bigger region based on previously recorded time-series data, which can help the farmers and common people to plan accordingly and take precautionary measures.Keywords
Cite This Article
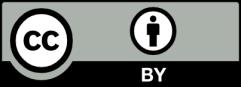
This work is licensed under a Creative Commons Attribution 4.0 International License , which permits unrestricted use, distribution, and reproduction in any medium, provided the original work is properly cited.