Open Access
ARTICLE
Enhancing Parkinson's Disease Prediction Using Machine Learning and Feature Selection Methods
1 College of Computer Science and Engineering, Taibah University, Medina, 41477, Saudi Arabia
2 School of Computing and Digital Technology, Birmingham City University, Birmingham, B47XG, United Kingdom
3 Information System Department, Saba'a Region University, Mareeb, Yemen
4 Computers and Systems Engineering Department, Al-Azhar University, Cairo, 11884, Egypt
5 RIADI Laboratory, National School of Computer Sciences, University of Manouba, Manouba, 2010, Tunisia
* Corresponding Author: Faisal Saeed. Email:
(This article belongs to the Special Issue: Computational Models for Pro-Smart Environments in Data Science Assisted IoT Systems)
Computers, Materials & Continua 2022, 71(3), 5639-5658. https://doi.org/10.32604/cmc.2022.023124
Received 29 August 2021; Accepted 19 November 2021; Issue published 14 January 2022
Abstract
Several millions of people suffer from Parkinson's disease globally. Parkinson's affects about 1% of people over 60 and its symptoms increase with age. The voice may be affected and patients experience abnormalities in speech that might not be noticed by listeners, but which could be analyzed using recorded speech signals. With the huge advancements of technology, the medical data has increased dramatically, and therefore, there is a need to apply data mining and machine learning methods to extract new knowledge from this data. Several classification methods were used to analyze medical data sets and diagnostic problems, such as Parkinson's Disease (PD). In addition, to improve the performance of classification, feature selection methods have been extensively used in many fields. This paper aims to propose a comprehensive approach to enhance the prediction of PD using several machine learning methods with different feature selection methods such as filter-based and wrapper-based. The dataset includes 240 recodes with 46 acoustic features extracted from 3 voice recording replications for 80 patients. The experimental results showed improvements when wrapper-based features selection method was used with K-NN classifier with accuracy of 88.33%. The best obtained results were compared with other studies and it was found that this study provides comparable and superior results.Keywords
Cite This Article
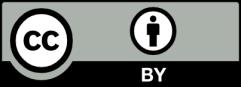
This work is licensed under a Creative Commons Attribution 4.0 International License , which permits unrestricted use, distribution, and reproduction in any medium, provided the original work is properly cited.