Open Access
ARTICLE
Fruit Image Classification Using Deep Learning
1 Department of Computer Science, Guru Arjan Dev Khalsa College Chohla Sahib, 143408, Punjab, India
2 Al-Nahrain University, Al-Nahrain Nano-Renewable Energy Research Center, Baghdad, 964, Iraq
3 Department of Computer Science, College of Computer and Information Systems, Umm Al-Qura University, Makkah, 21955, Saudi Arabia
4 Department of Information Technology, College of Computers and Information Technology, Taif University, Taif, 21944, Saudi Arabia
5 Department of Computer Engineering, College of Computers and Information Technology, Taif University, Taif, 21944, Saudi Arabia
* Corresponding Author: Harmandeep Singh Gill. Email:
Computers, Materials & Continua 2022, 71(3), 5135-5150. https://doi.org/10.32604/cmc.2022.022809
Received 19 August 2021; Accepted 10 November 2021; Issue published 14 January 2022
Abstract
Fruit classification is found to be one of the rising fields in computer and machine vision. Many deep learning-based procedures worked out so far to classify images may have some ill-posed issues. The performance of the classification scheme depends on the range of captured images, the volume of features, types of characters, choice of features from extracted features, and type of classifiers used. This paper aims to propose a novel deep learning approach consisting of Convolution Neural Network (CNN), Recurrent Neural Network (RNN), and Long Short-Term Memory (LSTM) application to classify the fruit images. Classification accuracy depends on the extracted and selected optimal features. Deep learning applications CNN, RNN, and LSTM were collectively involved to classify the fruits. CNN is used to extract the image features. RNN is used to select the extracted optimal features and LSTM is used to classify the fruits based on extracted and selected images features by CNN and RNN. Empirical study shows the supremacy of proposed over existing Support Vector Machine (SVM), Feed-forward Neural Network (FFNN), and Adaptive Neuro-Fuzzy Inference System (ANFIS) competitive techniques for fruit images classification. The accuracy rate of the proposed approach is quite better than the SVM, FFNN, and ANFIS schemes. It has been concluded that the proposed technique outperforms existing schemes.Keywords
Cite This Article
Citations
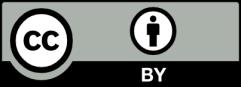
This work is licensed under a Creative Commons Attribution 4.0 International License , which permits unrestricted use, distribution, and reproduction in any medium, provided the original work is properly cited.