Open Access
ARTICLE
Skeleton Split Strategies for Spatial Temporal Graph Convolution Networks
Electronic and Electrical Engineering Department, University College London, London, WC1E 7JE, England
* Corresponding Author: Motasem S. Alsawadi. Email:
Computers, Materials & Continua 2022, 71(3), 4643-4658. https://doi.org/10.32604/cmc.2022.022783
Received 18 August 2021; Accepted 24 September 2021; Issue published 14 January 2022
Abstract
Action recognition has been recognized as an activity in which individuals’ behaviour can be observed. Assembling profiles of regular activities such as activities of daily living can support identifying trends in the data during critical events. A skeleton representation of the human body has been proven to be effective for this task. The skeletons are presented in graphs form-like. However, the topology of a graph is not structured like Euclidean-based data. Therefore, a new set of methods to perform the convolution operation upon the skeleton graph is proposed. Our proposal is based on the Spatial Temporal-Graph Convolutional Network (ST-GCN) framework. In this study, we proposed an improved set of label mapping methods for the ST-GCN framework. We introduce three split techniques (full distance split, connection split, and index split) as an alternative approach for the convolution operation. The experiments presented in this study have been trained using two benchmark datasets: NTU-RGB + D and Kinetics to evaluate the performance. Our results indicate that our split techniques outperform the previous partition strategies and are more stable during training without using the edge importance weighting additional training parameter. Therefore, our proposal can provide a more realistic solution for real-time applications centred on daily living recognition systems activities for indoor environments.Keywords
Cite This Article
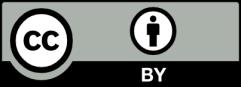
This work is licensed under a Creative Commons Attribution 4.0 International License , which permits unrestricted use, distribution, and reproduction in any medium, provided the original work is properly cited.