Open Access
ARTICLE
Design of Intelligent Alzheimer Disease Diagnosis Model on CIoT Environment
1 Department of Computer and Self Development, Preparatory Year Deanship, Prince Sattam bin Abdulaziz University, Al-Kharj, 16278, Saudi Arabia
2 Department of Computer Science, King King Khalid University, Muhayel Aseer, 62529, Saudi Arabia
3 Faculty of Computer and IT, Sana'a University, Sana'a, 61101, Yemen
4 Department of Computer Science, College of Computer, Qassim University, Al-Bukairiyah, 52571, Saudi Arabia
5 Department of Information Systems, College of Computer and Information Systems, Prince Sultan University, Saudi Arabia
6 Department of Information Systems, King King Khalid University, Muhayel Aseer, 62529, Saudi Arabia
7 Department of Natural and Applied Sciences, College of Community-Aflaj, Prince Sattam bin Abdulaziz University, Al-Kharj, 16278, Saudi Arabia
* Corresponding Author: Manar Ahmed Hamza. Email:
Computers, Materials & Continua 2022, 71(3), 5979-5994. https://doi.org/10.32604/cmc.2022.022686
Received 15 August 2021; Accepted 13 December 2021; Issue published 14 January 2022
Abstract
Presently, cognitive Internet of Things (CIoT) with cloud computing (CC) enabled intelligent healthcare models are developed, which enables communication with intelligent devices, sensor modules, and other stakeholders in the healthcare sector to avail effective decision making. On the other hand, Alzheimer disease (AD) is an advanced and degenerative illness which injures the brain cells, and its earlier detection is necessary for suitable interference by healthcare professional. In this aspect, this paper presents a new Oriented Features from Accelerated Segment Test (FAST) with Rotated Binary Robust Independent Elementary Features (BRIEF) Detector (ORB) with optimal artificial neural network (ORB-OANN) model for AD diagnosis and classification on the CIoT based smart healthcare system. For initial pre-processing, bilateral filtering (BLF) based noise removal and region of interest (RoI) detection processes are carried out. In addition, the ORB-OANN model includes ORB based feature extractor and principal component analysis (PCA) based feature selector. Moreover, artificial neural network (ANN) model is utilized as a classifier and the parameters of the ANN are optimally chosen by the use of salp swarm algorithm (SSA). A comprehensive experimental analysis of the ORB-OANN model is carried out on the benchmark database and the obtained results pointed out the promising outcome of the ORB-OANN technique in terms of different measures.Keywords
Cite This Article
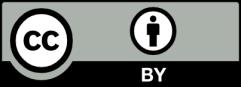
This work is licensed under a Creative Commons Attribution 4.0 International License , which permits unrestricted use, distribution, and reproduction in any medium, provided the original work is properly cited.