Open Access
ARTICLE
Gauss Gradient and SURF Features for Landmine Detection from GPR Images
1 Department of Electronics and Communications Engineering, Faculty of Engineering, Zagazig University, Zagazig, Sharqia, 44519, Egypt
2 Department of Electronics and Electrical Communications Engineering, Faculty of Electronic Engineering, Menoufia University, Menoufia, 32952, Egypt
3 Department of Industrial Electronics and Control Engineering, Faculty of Electronic Engineering, Menoufia University, Menouf, 32952, Egypt
4 Department of Information Technology, College of Computer and Information Sciences, Princess Nourah Bint Abdulrahman University, Riyadh, Saudi Arabia
* Corresponding Author: Abeer D. Algarni. Email:
Computers, Materials & Continua 2022, 71(3), 4457-4487. https://doi.org/10.32604/cmc.2022.022328
Received 04 August 2021; Accepted 15 September 2021; Issue published 14 January 2022
Abstract
Recently, ground-penetrating radar (GPR) has been extended as a well-known area to investigate the subsurface objects. However, its output has a low resolution, and it needs more processing for more interpretation. This paper presents two algorithms for landmine detection from GPR images. The first algorithm depends on a multi-scale technique. A Gaussian kernel with a particular scale is convolved with the image, and after that, two gradients are estimated; horizontal and vertical gradients. Then, histogram and cumulative histogram are estimated for the overall gradient image. The bin values on the cumulative histogram are used for discrimination between images with and without landmines. Moreover, a neural classifier is used to classify images with cumulative histograms as feature vectors. The second algorithm is based on scale-space analysis with the number of speeded-up robust feature (SURF) points as the key parameter for classification. In addition, this paper presents a framework for size reduction of GPR images based on decimation for efficient storage. The further classification steps can be performed on images after interpolation. The sensitivity of classification accuracy to the interpolation process is studied in detail.Keywords
Cite This Article
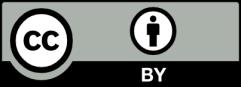
This work is licensed under a Creative Commons Attribution 4.0 International License , which permits unrestricted use, distribution, and reproduction in any medium, provided the original work is properly cited.