Open Access
ARTICLE
An Optimized Convolution Neural Network Architecture for Paddy Disease Classification
1 Faculty of Computer Science, Universiti Tun Hussein Onn Malaysia, Batu Pahat, 54000, Malaysia
2 School of Electronics, Computing and Mathematics, University of Derby, Derby, DE22 1GB, United Kingdom
3 Department of Computer Science, Lahore Garrison University, Lahore, 54000, Pakistan
* Corresponding Author: Muhammad Aamir. Email:
(This article belongs to the Special Issue: Application of Machine-Learning in Computer Vision)
Computers, Materials & Continua 2022, 71(3), 6053-6067. https://doi.org/10.32604/cmc.2022.022215
Received 31 July 2021; Accepted 16 November 2021; Issue published 14 January 2022
Abstract
Plant disease classification based on digital pictures is challenging. Machine learning approaches and plant image categorization technologies such as deep learning have been utilized to recognize, identify, and diagnose plant diseases in the previous decade. Increasing the yield quantity and quality of rice forming is an important cause for the paddy production countries. However, some diseases that are blocking the improvement in paddy production are considered as an ominous threat. Convolution Neural Network (CNN) has shown a remarkable performance in solving the early detection of paddy leaf diseases based on its images in the fast-growing era of science and technology. Nevertheless, the significant CNN architectures construction is dependent on expertise in a neural network and domain knowledge. This approach is time-consuming, and high computational resources are mandatory. In this research, we propose a novel method based on Mutant Particle swarm optimization (MUT-PSO) Algorithms to search for an optimum CNN architecture for Paddy leaf disease classification. Experimentation results show that Mutant Particle swarm optimization Convolution Neural Network (MUTPSO-CNN) can find optimum CNN architecture that offers better performance than existing hand-crafted CNN architectures in terms of accuracy, precision/recall, and execution time.Keywords
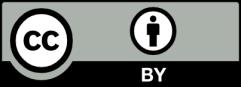