Open Access
ARTICLE
Parking Availability Prediction with Coarse-Grained Human Mobility Data
1 University of Murcia, Murcia, 30003, Spain
2 Universidad Católica de Murcia (UCAM), Murcia, 30107, Spain
* Corresponding Author: Fernando Terroso-Sáenz. Email:
Computers, Materials & Continua 2022, 71(3), 4355-4375. https://doi.org/10.32604/cmc.2022.021492
Received 04 July 2021; Accepted 14 October 2021; Issue published 14 January 2022
Abstract
Nowadays, the anticipation of parking-space demand is an instrumental service in order to reduce traffic congestion levels in urban spaces. The purpose of our work is to study, design and develop a parking-availability predictor that extracts the knowledge from human mobility data, based on the anonymized human displacements of an urban area, and also from weather conditions. Most of the existing solutions for this prediction take as contextual data the current road-traffic state defined at very high temporal or spatial resolution. However, access to this type of fine-grained location data is usually quite limited due to several economic or privacy-related restrictions. To overcome this limitation, our proposal uses urban areas that are defined at very low spatial and temporal resolution. We conducted several experiments using three Artificial Neural Networks: Multilayer Perceptron, Gated Recurrent Units and bidirectional Long Short Term Memory networks and we tested their suitability using different combinations of inputs. Several metrics are provided for the sake of comparison within our study and between other studies. The solution has been evaluated in a real-world testbed in the city of Murcia (Spain) integrating an open human-mobility dataset showing high accuracy. A MAPE between 4% and 10% was reported in horizons of 1 to 3 h.Keywords
Cite This Article
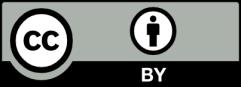