Open Access
ARTICLE
Modified UNet Model for Brain Stroke Lesion Segmentation on Computed Tomography Images
1 Al-Farabi Kazakh National University, Almaty, Kazakhstan
2 International University of Tourism and Hospitality, Turkistan, Kazakhstan
3 Khoja Akhmet Yassawi International Kazakh-Turkish University, Turkistan, Kazakhstan
4 Instituto Universitário de Lisboa, Lisbon, Portugal
5 International Information Technology University, Almaty, Kazakhstan
6 South Kazakhstan State Pedagogical University, Shymkent, Kazakhstan
7 M. Auezov South Kazakhstan University, Shymkent, Kazakshtan
8 Kazakh-British Technical University, Almaty, Kazakhstan
9 Asfendiyarov Kazakh National Medical University, Almaty, Kazakhstan
10 Kazakh National Women's Teacher Training University, Almaty, Kazakhstan
11 Abai Kazakh National Pedagogical University, Almaty, Kazakhstan
* Corresponding Author: Azhar Tursynova. Email:
Computers, Materials & Continua 2022, 71(3), 4701-4717. https://doi.org/10.32604/cmc.2022.020998
Received 18 June 2021; Accepted 22 September 2021; Issue published 14 January 2022
Abstract
The task of segmentation of brain regions affected by ischemic stroke is help to tackle important challenges of modern stroke imaging analysis. Unfortunately, at the moment, the models for solving this problem using machine learning methods are far from ideal. In this paper, we consider a modified 3D UNet architecture to improve the quality of stroke segmentation based on 3D computed tomography images. We use the ISLES 2018 (Ischemic Stroke Lesion Segmentation Challenge 2018) open dataset to train and test the proposed model. Interpretation of the obtained results, as well as the ideas for further experiments are included in the paper. Our evaluation is performed using the Dice or f1 score coefficient and the Jaccard index. Our architecture may simply be extended to ischemia segmentation and computed tomography image identification by selecting relevant hyperparameters. The Dice/f1 score similarity coefficient of our model shown 58% and results close to ground truth which is higher than the standard 3D UNet model, demonstrating that our model can accurately segment ischemic stroke. The modified 3D UNet model proposed by us uses an efficient averaging method inside a neural network. Since this set of ISLES is limited in number, using the data augmentation method and neural network regularization methods to prevent overfitting gave the best result. In addition, one of the advantages is the use of the Intersection over Union loss function, which is based on the assessment of the coincidence of the shapes of the recognized zones.Keywords
Cite This Article
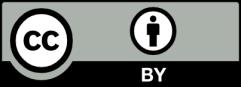