Open Access
ARTICLE
Machine Learning-Based Predictions on the Self-Heating Characteristics of Nanocomposites with Hybrid Fillers
1 Department of Civil and Environmental Engineering, Korea Advanced Institute of Science and Technology (KAIST), Daejeon, 34141, Korea
2 School of Civil Engineering, Chungbuk National University, Cheongju, 28644, Korea
* Corresponding Author: Beomjoo Yang. Email:
Computers, Materials & Continua 2022, 71(3), 4487-4502. https://doi.org/10.32604/cmc.2022.020940
Received 15 June 2021; Accepted 23 September 2021; Issue published 14 January 2022
Abstract
A machine learning-based prediction of the self-heating characteristics and the negative temperature coefficient (NTC) effect detection of nanocomposites incorporating carbon nanotube (CNT) and carbon fiber (CF) is proposed. The CNT content was fixed at 4.0 wt.%, and CFs having three different lengths (0.1, 3 and 6 mm) at dosage of 1.0 wt.% were added to fabricate the specimens. The self-heating properties of the specimens were evaluated via self-heating tests. Based on the experiment results, two types of artificial neural network (ANN) models were constructed to predict the surface temperature and electrical resistance, and to detect a severe NTC effect. The present predictions were compared with experimental values to verify the applicability of the proposed ANN models. The ANN model for data prediction was able to predict the surface temperature and electrical resistance closely, with corresponding R-squared value of 0.91 and 0.97, respectively. The ANN model for data detection could detect the severe NTC effect occurred in the nanocomposites under the self-heating condition, as evidenced by the accuracy and sensitivity values exceeding 0.7 in all criteria.Keywords
Cite This Article
Citations
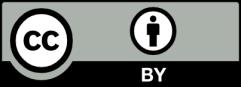