Open Access
ARTICLE
Hybrid In-Vehicle Background Noise Reduction for Robust Speech Recognition: The Possibilities of Next Generation 5G Data Networks
1 VSB–Technical University of Ostrava, Faculty of Electrical Engineering and Computer Science, Department of Cybernetics and Biomedical Engineering, 708 00, Ostrava-Poruba, Czechia
2 VSB–Technical University of Ostrava, Faculty of Electrical Engineering and Computer Science, Department of Telecommunications, 708 00, Ostrava-Poruba, Czechia
* Corresponding Author: Lukas Danys. Email:
Computers, Materials & Continua 2022, 71(3), 4659-4676. https://doi.org/10.32604/cmc.2022.019904
Received 30 April 2021; Accepted 01 July 2021; Issue published 14 January 2022
Abstract
This pilot study focuses on employment of hybrid LMS-ICA system for in-vehicle background noise reduction. Modern vehicles are nowadays increasingly supporting voice commands, which are one of the pillars of autonomous and SMART vehicles. Robust speaker recognition for context-aware in-vehicle applications is limited to a certain extent by in-vehicle background noise. This article presents the new concept of a hybrid system, which is implemented as a virtual instrument. The highly modular concept of the virtual car used in combination with real recordings of various driving scenarios enables effective testing of the investigated methods of in-vehicle background noise reduction. The study also presents a unique concept of an adaptive system using intelligent clusters of distributed next generation 5G data networks, which allows the exchange of interference information and/or optimal hybrid algorithm settings between individual vehicles. On average, the unfiltered voice commands were successfully recognized in 29.34% of all scenarios, while the LMS reached up to 71.81%, and LMS-ICA hybrid improved the performance further to 73.03%.Keywords
Cite This Article
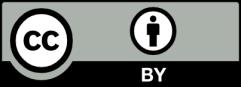