Open Access
ARTICLE
VANET Jamming and Adversarial Attack Defense for Autonomous Vehicle Safety
1 Department of Vehicle Convergence Engineering, Yonsei University, Seoul, 03722, Korea
2 School of Electrical & Electronic Engineering, Yonsei University, Seoul, 03722, Korea
* Corresponding Author: Jong-Moon Chung. Email:
Computers, Materials & Continua 2022, 71(2), 3589-3605. https://doi.org/10.32604/cmc.2022.023073
Received 27 August 2021; Accepted 13 October 2021; Issue published 07 December 2021
Abstract
The development of Vehicular Ad-hoc Network (VANET) technology is helping Intelligent Transportation System (ITS) services to become a reality. Vehicles can use VANETs to communicate safety messages on the road (while driving) and can inform their location and share road condition information in real-time. However, intentional and unintentional (e.g., packet/frame collision) wireless signal jamming can occur, which will degrade the quality of communication over the channel, preventing the reception of safety messages, and thereby posing a safety hazard to the vehicle's passengers. In this paper, VANET jamming detection applying Support Vector Machine (SVM) machine learning technology is used to classify jamming and non-jamming situations. The analysis is based on two cases which include normal traffic and heavy traffic conditions, where the results show that the probability of packet dropping will increase when many vehicles are using the wireless channel simultaneously. When using SVM classification, the most appropriate feature set applied in determining a jamming situation shows an accuracy of 98% or higher. Furthermore, more advanced jamming attacks need to be considered for preparation of more reliable and safer autonomous ITS services. Such research can use vehicular communication transmission and reception data based on selected published datasets. In this paper, an additional adversarial defense algorithm using the Density-Based Spatial Clustering of Applications with Noise (DBSCAN) method is proposed, which assumes that evolutionary attacks of the jammer will attempt to confuse the trained classifier. The simulation results show that applying DBSCAN can improve the accuracy by elimination of outliers before conducting classification testing.Keywords
Cite This Article
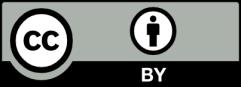