Open Access
ARTICLE
CDLSTM: A Novel Model for Climate Change Forecasting
Department of Computer Science, College of Computer and Information Sciences, Majmaah University, AL Majmaah, 11952, Saudi Arabia
* Corresponding Author: Mohd Anul Haq. Email:
(This article belongs to the Special Issue: Artificial Intelligence and Machine Learning Algorithms in Real-World Applications and Theories)
Computers, Materials & Continua 2022, 71(2), 2363-2381. https://doi.org/10.32604/cmc.2022.023059
Received 26 August 2021; Accepted 27 September 2021; Issue published 07 December 2021
Abstract
Water received in rainfall is a crucial natural resource for agriculture, the hydrological cycle, and municipal purposes. The changing rainfall pattern is an essential aspect of assessing the impact of climate change on water resources planning and management. Climate change affected the entire world, specifically India’s fragile Himalayan mountain region, which has high significance due to being a climatic indicator. The water coming from Himalayan rivers is essential for 1.4 billion people living downstream. Earlier studies either modeled temperature or rainfall for the Himalayan area; however, the combined influence of both in a long-term analysis was not performed utilizing Deep Learning (DL). The present investigation attempted to analyze the time series and correlation of temperature (1796–2013) and rainfall changes (1901–2015) over the Himalayan states in India. The Climate Deep Long Short-Term Memory (CDLSTM) model was developed and optimized to forecast all Himalayan states’ temperature and rainfall values. Facebook’s Prophet (FB-Prophet) model was implemented to forecast and assess the performance of the developed CDLSTM model. The performance of both models was assessed based on various performance metrics and shown significantly higher accuracies and low error rates.Keywords
Cite This Article
Citations
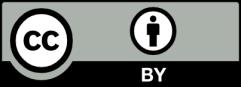
This work is licensed under a Creative Commons Attribution 4.0 International License , which permits unrestricted use, distribution, and reproduction in any medium, provided the original work is properly cited.