Open Access
ARTICLE
Multi-Scale Image Segmentation Model for Fine-Grained Recognition of Zanthoxylum Rust
1 School of Information and Communication Engineering, University of Electronic Science and Technology of China, Chengdu, 611731, China
2 College of Forestry, Sichuan Agricultural University, Chengdu, 611130, China
3 Sichuan Zizhou Agricultural Science and Technology Co. Ltd., Mianyang, 621100, China
4 Department of Electronic and Computer Engineering, Brunel University, Uxbridge, Middlesex, UB8 3PH, United Kingdom
* Corresponding Author: Jie Xu. Email:
Computers, Materials & Continua 2022, 71(2), 2963-2980. https://doi.org/10.32604/cmc.2022.022810
Received 19 August 2021; Accepted 09 October 2021; Issue published 07 December 2021
Abstract
Zanthoxylum bungeanum Maxim, generally called prickly ash, is widely grown in China. Zanthoxylum rust is the main disease affecting the growth and quality of Zanthoxylum. Traditional method for recognizing the degree of infection of Zanthoxylum rust mainly rely on manual experience. Due to the complex colors and shapes of rust areas, the accuracy of manual recognition is low and difficult to be quantified. In recent years, the application of artificial intelligence technology in the agricultural field has gradually increased. In this paper, based on the DeepLabV2 model, we proposed a Zanthoxylum rust image segmentation model based on the FASPP module and enhanced features of rust areas. This paper constructed a fine-grained Zanthoxylum rust image dataset. In this dataset, the Zanthoxylum rust image was segmented and labeled according to leaves, spore piles, and brown lesions. The experimental results showed that the Zanthoxylum rust image segmentation method proposed in this paper was effective. The segmentation accuracy rates of leaves, spore piles and brown lesions reached 99.66%, 85.16% and 82.47% respectively. MPA reached 91.80%, and MIoU reached 84.99%. At the same time, the proposed image segmentation model also had good efficiency, which can process 22 images per minute. This article provides an intelligent method for efficiently and accurately recognizing the degree of infection of Zanthoxylum rust.Keywords
Cite This Article
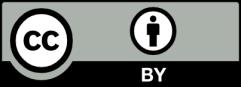
This work is licensed under a Creative Commons Attribution 4.0 International License , which permits unrestricted use, distribution, and reproduction in any medium, provided the original work is properly cited.