Open Access
ARTICLE
Deep Learning Based Automated Diagnosis of Skin Diseases Using Dermoscopy
1 Chitkara University Institute of Engineering and Technology, Chitkara University, Punjab, India
2 Department of Systemics, School of Computer Science, University of Petroleum & Energy Studies, Dehradun, Uttrakhand, India
3 School of Electronics & Communication, Shri Mata Vaishno Devi University, Katra, 182320, India
4 Department of Computer Science, College of Computers and Information Technology, Taif University, Taif, 21944, Saudi Arabia
* Corresponding Author: Deepika Koundal. Email:
(This article belongs to the Special Issue: Applications of Intelligent Systems in Computer Vision)
Computers, Materials & Continua 2022, 71(2), 3145-3160. https://doi.org/10.32604/cmc.2022.022788
Received 18 August 2021; Accepted 15 October 2021; Issue published 07 December 2021
Abstract
Biomedical image analysis has been exploited considerably by recent technology involvements, carrying about a pattern shift towards ‘automation’ and ‘error free diagnosis’ classification methods with markedly improved accurate diagnosis productivity and cost effectiveness. This paper proposes an automated deep learning model to diagnose skin disease at an early stage by using Dermoscopy images. The proposed model has four convolutional layers, two maxpool layers, one fully connected layer and three dense layers. All the convolutional layers are using the kernel size of 3 * 3 whereas the maxpool layer is using the kernel size of 2 * 2. The dermoscopy images are taken from the HAM10000 dataset. The proposed model is compared with the three different models of ResNet that are ResNet18, ResNet50 and ResNet101. The models are simulated with 32 batch size and Adadelta optimizer. The proposed model has obtained the best accuracy value of 0.96 whereas the ResNet101 model has obtained 0.90, the ResNet50 has obtained 0.89 and the ResNet18 model has obtained value as 0.86. Therefore, features obtained from the proposed model are more capable for improving the classification performance of multiple skin disease classes. This model can be used for early diagnosis of skin disease and can also act as a second opinion tool for dermatologists.Keywords
Cite This Article
Citations
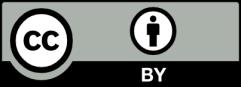