Open Access
ARTICLE
Automated Grading of Breast Cancer Histopathology Images Using Multilayered Autoencoder
1 Knowledge Unit of System and Technology, University of Management & Technology, Sialkot Campus, 51310, Pakistan
2 Department of Computer Science, University of Management and Technology, Sialkot Campus, 51310, Pakistan
3 Faculty of Computing and Technology, University of Engineering & Technology, Narowal, 51610, Pakistan
4 College of Computer Science and Engineering, University of Jeddah, 21493, Saudi Arabia
5 Faculty of Computing and Information Technology, King Abdulaziz University, Jeddah, 21589, Saudi Arabia
6 Department of Computer Engineering, College of Computers and Information Technology, Taif University, Taif, Saudi Arabia
7 Faculty of Engineering, Moncton University, NB, E1A3E9, Canada
8 Department of Information and Communication Engineering, Yeungnam University, Gyeongsan, 38541, Korea
* Corresponding Author: Muhammad Shafiq. Email:
Computers, Materials & Continua 2022, 71(2), 3407-3423. https://doi.org/10.32604/cmc.2022.022705
Received 16 August 2021; Accepted 30 September 2021; Issue published 07 December 2021
Abstract
Breast cancer (BC) is the most widely recognized cancer in women worldwide. By 2018, 627,000 women had died of breast cancer (World Health Organization Report 2018). To diagnose BC, the evaluation of tumours is achieved by analysis of histological specimens. At present, the Nottingham Bloom Richardson framework is the least expensive approach used to grade BC aggressiveness. Pathologists contemplate three elements, 1. mitotic count, 2. gland formation, and 3. nuclear atypia, which is a laborious process that witness's variations in expert's opinions. Recently, some algorithms have been proposed for the detection of mitotic cells, but nuclear atypia in breast cancer histopathology has not received much consideration. Nuclear atypia analysis is performed not only to grade BC but also to provide critical information in the discrimination of normal breast, non-invasive breast (usual ductal hyperplasia, atypical ductal hyperplasia) and pre-invasive breast (ductal carcinoma in situ) and invasive breast lesions. We proposed a deep-stacked multi-layer autoencoder ensemble with a softmax layer for the feature extraction and classification process. The classification results show the value of the multi-layer autoencoder model in the evaluation of nuclear polymorphisms. The proposed method has indicated promising results, making them more fit in breast cancer grading.Keywords
Cite This Article
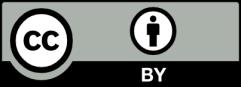
This work is licensed under a Creative Commons Attribution 4.0 International License , which permits unrestricted use, distribution, and reproduction in any medium, provided the original work is properly cited.