Open Access
ARTICLE
Modeling of Artificial Intelligence Based Traffic Flow Prediction with Weather Conditions
1 Department of Natural and Applied Sciences, College of Community-Aflaj, Prince Sattam Bin Abdulaziz University, Al-Kharj, 16278, Saudi Arabia
2 Department of Information Systems, College of Computer and Information Sciences, Princess Nourah Bint Abdulrahman University, Riyadh, 11564, Saudi Arabia
3 Department of Computer Science, College of Science & Arts at Mahayil, King Khalid University, Muhayel Aseer, 62529, Saudi Arabia
4 Faculty of Computer and IT, Sana'a University, Sana'a, 61101, Yemen
5 Department of Computer Science, College of Science and Arts in Al-Bukairiyah, Qassim University, Al-Bukairiyah, 52571, Saudi Arabia
6 Department of Information Systems, College of Science & Arts at Mahayil, King Khalid University, Muhayel Aseer, 62529, Saudi Arabia
7 Department of Computer and Self Development, Preparatory Year Deanship, Prince Sattam Bin Abdulaziz University, Al-Kharj, 16278, Saudi Arabia
* Corresponding Author: Manar Ahmed Hamza. Email:
Computers, Materials & Continua 2022, 71(2), 3953-3968. https://doi.org/10.32604/cmc.2022.022692
Received 16 August 2021; Accepted 16 September 2021; Issue published 07 December 2021
Abstract
Short-term traffic flow prediction (TFP) is an important area in intelligent transportation system (ITS), which is used to reduce traffic congestion. But the avail of traffic flow data with temporal features and periodic features are susceptible to weather conditions, making TFP a challenging issue. TFP process are significantly influenced by several factors like accident and weather. Particularly, the inclement weather conditions may have an extreme impact on travel time and traffic flow. Since most of the existing TFP techniques do not consider the impact of weather conditions on the TF, it is needed to develop effective TFP with the consideration of extreme weather conditions. In this view, this paper designs an artificial intelligence based TFP with weather conditions (AITFP-WC) for smart cities. The goal of the AITFP-WC model is to enhance the performance of the TFP model with the inclusion of weather related conditions. The proposed AITFP-WC technique includes Elman neural network (ENN) model to predict the flow of traffic in smart cities. Besides, tunicate swarm algorithm with feed forward neural networks (TSA-FFNN) model is employed for the weather and periodicity analysis. At last, a fusion of TFP and WPA processes takes place using the FFNN model to determine the final prediction output. In order to assess the enhanced predictive outcome of the AITFP-WC model, an extensive simulation analysis is carried out. The experimental values highlighted the enhanced performance of the AITFP-WC technique over the recent state of art methods.Keywords
Cite This Article
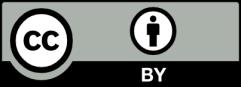