Open Access
ARTICLE
Diabetic Retinopathy Detection Using Classical-Quantum Transfer Learning Approach and Probability Model
1 Department of Physics, Comsats University Islamabad, Lahore Campus, Lahore, 54000, Pakistan
2 Department of Statistics, Comsats University Islamabad, Lahore Campus, Lahore, 54000, Pakistan
3 Department of Computer Science, Comsats University Islamabad, Lahore Campus, Lahore, 54000, Pakistan
* Corresponding Author: Atifa Athar. Email:
(This article belongs to the Special Issue: Advances in Artificial Intelligence and Machine learning in Biomedical and Healthcare Informatics)
Computers, Materials & Continua 2022, 71(2), 3733-3746. https://doi.org/10.32604/cmc.2022.022524
Received 10 August 2021; Accepted 19 October 2021; Issue published 07 December 2021
Abstract
Diabetic Retinopathy (DR) is a common complication of diabetes mellitus that causes lesions on the retina that affect vision. Late detection of DR can lead to irreversible blindness. The manual diagnosis process of DR retina fundus images by ophthalmologists is time consuming and costly. While, Classical Transfer learning models are extensively used for computer aided detection of DR; however, their maintenance costs limits detection performance rate. Therefore, Quantum Transfer learning is a better option to address this problem in an optimized manner. The significance of Hybrid quantum transfer learning approach includes that it performs heuristically. Thus, our proposed methodology aims to detect DR using a hybrid quantum transfer learning approach. To build our model we extract the APTOS 2019 Blindness Detection dataset from Kaggle and used inception-V3 pre-trained classical neural network for feature extraction and Variational Quantum classifier for stratification and trained our model on Penny Lane default device, IBM Qiskit BasicAer device and Google Cirq Simulator device. Both models are built based on PyTorch machine learning library. We bring about a contrast performance rate between classical and quantum models. Our proposed model achieves an accuracy of 93%–96% on the quantum hybrid model and 85% accuracy rate on the classical model. So, quantum computing can harness quantum machine learning to do work with power and efficiency that is not possible for classical computers.Keywords
Cite This Article
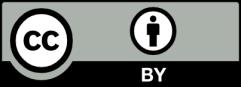