Open Access
ARTICLE
Deep Neural Network and Pseudo Relevance Feedback Based Query Expansion
Department of Mathematics, Bio-Informatics and Computer Applications, Maulana Azad National Institute of Technology Bhopal, Bhopal, Madhya Pradesh, 462003, India
* Corresponding Author: Abhishek Kumar Shukla. Email:
(This article belongs to the Special Issue: Emerging Applications of Artificial Intelligence, Machine learning and Data Science)
Computers, Materials & Continua 2022, 71(2), 3557-3570. https://doi.org/10.32604/cmc.2022.022411
Received 06 August 2021; Accepted 13 September 2021; Issue published 07 December 2021
Abstract
The neural network has attracted researchers immensely in the last couple of years due to its wide applications in various areas such as Data mining, Natural language processing, Image processing, and Information retrieval etc. Word embedding has been applied by many researchers for Information retrieval tasks. In this paper word embedding-based skip-gram model has been developed for the query expansion task. Vocabulary terms are obtained from the top “k” initially retrieved documents using the Pseudo relevance feedback model and then they are trained using the skip-gram model to find the expansion terms for the user query. The performance of the model based on mean average precision is 0.3176. The proposed model compares with other existing models. An improvement of 6.61%, 6.93%, and 9.07% on MAP value is observed compare to the Original query, BM25 model, and query expansion with the Chi-Square model respectively. The proposed model also retrieves 84, 25, and 81 additional relevant documents compare to the original query, query expansion with Chi-Square model, and BM25 model respectively and thus improves the recall value also. The per query analysis reveals that the proposed model performs well in 30, 36, and 30 queries compare to the original query, query expansion with Chi-square model, and BM25 model respectively.Keywords
Cite This Article
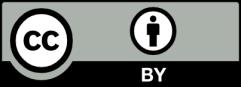
This work is licensed under a Creative Commons Attribution 4.0 International License , which permits unrestricted use, distribution, and reproduction in any medium, provided the original work is properly cited.