Open Access
ARTICLE
A Deep Two-State Gated Recurrent Unit for Particulate Matter (PM2.5) Concentration Forecasting
1 Faculty of Computer Science and Information Technology, Universiti Tun Hussein Onn Malaysia, Batu Pahat, Johor, Malaysia
2 College of Computer Science, King Khalid University, Abha, Saudi Arabia
3 College of Computer and Information Sciences, Imam Mohammad Ibn Saud Islamic University, Riyadh, Saudi Arabia
4 Department of Management Information Systems, College of Business Administration, Imam Abdulrahman Bin Faisal University, 31441, Dammam, Saudi Arabia
* Corresponding Author: Rozaida Ghazali. Email:
(This article belongs to the Special Issue: Artificial Intelligence and Machine Learning Algorithms in Real-World Applications and Theories)
Computers, Materials & Continua 2022, 71(2), 3051-3068. https://doi.org/10.32604/cmc.2022.021629
Received 08 July 2021; Accepted 23 August 2021; Issue published 07 December 2021
Abstract
Air pollution is a significant problem in modern societies since it has a serious impact on human health and the environment. Particulate Matter (PM2.5) is a type of air pollution that contains of interrupted elements with a diameter less than or equal to 2.5 m. For risk assessment and epidemiological investigations, a better knowledge of the spatiotemporal variation of PM2.5 concentration in a constant space-time area is essential. Conventional spatiotemporal interpolation approaches commonly relying on robust presumption by limiting interpolation algorithms to those with explicit and basic mathematical expression, ignoring a plethora of hidden but crucial manipulating aspects. Many advanced deep learning approaches have been proposed to forecast Particulate Matter (PM2.5). Recurrent neural network (RNN) is one of the popular deep learning architectures which is widely employed in PM2.5 concentration forecasting. In this research, we proposed a Two-State Gated Recurrent Unit (TS-GRU) for monitoring and estimating the PM2.5 concentration forecasting system. The proposed algorithm is capable of considering both spatial and temporal hidden affecting elements spontaneously. We tested our model using data from daily PM2.5 dimensions taken in the contactual southeast area of the United States in 2009. In the studies, three evaluation matrices were utilized to compare the overall performance of each algorithm: Mean Absolute Error (MAE), Root Mean Square Error (RMSE), and Mean Absolute Percentage Error (MAPE). The experimental results revealed that our proposed TS-GRU model outperformed compared to the other deep learning approaches in terms of forecasting performance.Keywords
Cite This Article
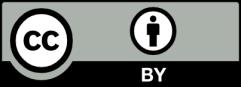