Open Access
ARTICLE
A DQN-Based Cache Strategy for Mobile Edge Networks
1 School of Electronic Engineering, Beijing University of Posts and Telecommunications, Beijing, 100876, China
2 State Key Laboratory of Intelligent Manufacturing System Technology, Beijing Institute of Electronic System Engineering, Beijing, 100854, China
3 Ningbo Sunny Intelligent Technology Co., LTD, Yuyao, 315400, China
4 Dublin City University, Dublin 9, Ireland
* Corresponding Author: Siyuan Sun. Email:
Computers, Materials & Continua 2022, 71(2), 3277-3291. https://doi.org/10.32604/cmc.2022.020471
Received 25 May 2021; Accepted 11 July 2021; Issue published 07 December 2021
Abstract
The emerging mobile edge networks with content caching capability allows end users to receive information from adjacent edge servers directly instead of a centralized data warehouse, thus the network transmission delay and system throughput can be improved significantly. Since the duplicate content transmissions between edge network and remote cloud can be reduced, the appropriate caching strategy can also improve the system energy efficiency of mobile edge networks to a great extent. This paper focuses on how to improve the network energy efficiency and proposes an intelligent caching strategy according to the cached content distribution model for mobile edge networks based on promising deep reinforcement learning algorithm. The deep neural network (DNN) and Q-learning algorithm are combined to design a deep reinforcement learning framework named as the deep-Q neural network (DQN), in which the DNN is adopted to represent the approximation of action-state value function in the Q-learning solution. The parameters iteration strategies in the proposed DQN algorithm were improved through stochastic gradient descent method, so the DQN algorithm could converge to the optimal solution quickly, and the network performance of the content caching policy can be optimized. The simulation results show that the proposed intelligent DQN-based content cache strategy with enough training steps could improve the energy efficiency of the mobile edge networks significantly.Keywords
Cite This Article
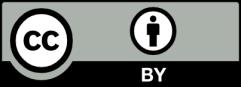