Open Access
ARTICLE
Generating A New Shilling Attack for Recommendation Systems
1 Department of Computer Science & Engineering, National Institute of Technology, Durgapur, India
2 Graduate School, Duy Tan University, Da Nang, Vietnam
3 Faculty of Information Technology, Duy Tan University, Da Nang, Vietnam
4 Department of Computer Science, College of Computers and Information Technology, Taif University, Taif, 21944, Saudi Arabia
* Corresponding Author: Anand Nayyar. Email:
Computers, Materials & Continua 2022, 71(2), 2827-2846. https://doi.org/10.32604/cmc.2022.020437
Received 24 May 2021; Accepted 27 June 2021; Issue published 07 December 2021
Abstract
A collaborative filtering-based recommendation system has been an integral part of e-commerce and e-servicing. To keep the recommendation systems reliable, authentic, and superior, the security of these systems is very crucial. Though the existing shilling attack detection methods in collaborative filtering are able to detect the standard attacks, in this paper, we prove that they fail to detect a new or unknown attack. We develop a new attack model, named Obscure attack, with unknown features and observed that it has been successful in biasing the overall top-N list of the target users as intended. The Obscure attack is able to push target items to the top-N list as well as remove the actual rated items from the list. Our proposed attack is more effective at a smaller number of k in top-k similar user as compared to other existing attacks. The effectivity of the proposed attack model is tested on the MovieLens dataset, where various classifiers like SVM, J48, random forest, and naïve Bayes are utilized.Keywords
Cite This Article
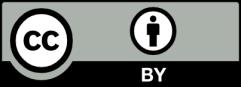
This work is licensed under a Creative Commons Attribution 4.0 International License , which permits unrestricted use, distribution, and reproduction in any medium, provided the original work is properly cited.