Open Access
ARTICLE
Blood Pressure and Heart Rate Measurements Using Photoplethysmography with Modified LRCN
1 Department of Electrical Engineering, National Taiwan Ocean University, Keelung City, 202301, Taiwan
2 Department of Electrical Engineering, National Formosa University, Yunlin County, 632, Taiwan
* Corresponding Author: Chih-Ta Yen. Email:
Computers, Materials & Continua 2022, 71(1), 1973-1986. https://doi.org/10.32604/cmc.2022.022679
Received 15 August 2021; Accepted 18 September 2021; Issue published 03 November 2021
Abstract
In this study, single-channel photoplethysmography (PPG) signals were used to estimate the heart rate (HR), diastolic blood pressure (DBP), and systolic blood pressure (SBP). A deep learning model was proposed using a long-term recurrent convolutional network (LRCN) modified from a deep learning algorithm, the convolutional neural network model of the modified inception deep learning module, and a long short-term memory network (LSTM) to improve the model's accuracy of BP and HR measurements. The PPG data of 1,551 patients were obtained from the University of California Irvine Machine Learning Repository. How to design a filter of PPG signals and how to choose the loss functions for deep learning model were also discussed in the study. Finally, the stability of the proposed model was tested using a 10-fold cross-validation, with an MAE ± SD of 2.942 ± 5.076 mmHg for SBP, 1.747 ± 3.042 mmHg for DBP, and 1.137 ± 2.463 bpm for the HR. Compared with its existing counterparts, the model entailed less computational load and was more accurate in estimating SBP, DBP, and HR. These results established the validity of the model.Keywords
Cite This Article
Citations
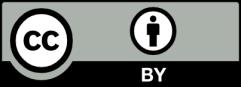
This work is licensed under a Creative Commons Attribution 4.0 International License , which permits unrestricted use, distribution, and reproduction in any medium, provided the original work is properly cited.