Open Access
ARTICLE
Hypo-Driver: A Multiview Driver Fatigue and Distraction Level Detection System
1 College of Computer and Information Sciences, Imam Mohammad Ibn Saud Islamic University (IMSIU), Riyadh, 11432, Saudi Arabia
2 Department of Electrical Engineering, Benha Faculty of Engineering, Benha University, Qalubia, Benha, 13518, Egypt
* Corresponding Author: Qaisar Abbas. Email:
(This article belongs to the Special Issue: Artificial Intelligence Enabled Intelligent Transportation Systems)
Computers, Materials & Continua 2022, 71(1), 1999-2007. https://doi.org/10.32604/cmc.2022.022553
Received 11 August 2021; Accepted 22 September 2021; Issue published 03 November 2021
Abstract
Traffic accidents are caused by driver fatigue or distraction in many cases. To prevent accidents, several low-cost hypovigilance (hypo-V) systems were developed in the past based on a multimodal-hybrid (physiological and behavioral) feature set. Similarly in this paper, real-time driver inattention and fatigue (Hypo-Driver) detection system is proposed through multi-view cameras and biosignal sensors to extract hybrid features. The considered features are derived from non-intrusive sensors that are related to the changes in driving behavior and visual facial expressions. To get enhanced visual facial features in uncontrolled environment, three cameras are deployed on multiview points (0°, 45°, and 90°) of the drivers. To develop a Hypo-Driver system, the physiological signals (electroencephalography (EEG), electrocardiography (ECG), electro-myography (sEMG), and electrooculography (EOG)) and behavioral information (PERCLOS70-80-90%, mouth aspect ratio (MAR), eye aspect ratio (EAR), blinking frequency (BF), head-titled ratio (HT-R)) are collected and pre-processed, then followed by feature selection and fusion techniques. The driver behaviors are classified into five stages such as normal, fatigue, visual inattention, cognitive inattention, and drowsy. This improved hypo-Driver system utilized trained behavioral features by a convolutional neural network (CNNs), recurrent neural network and long short-term memory (RNN-LSTM) model is used to extract physiological features. After fusion of these features, the Hypo-Driver system is classified hypo-V into five stages based on trained layers and dropout-layer in the deep-residual neural network (DRNN) model. To test the performance of a hypo-Driver system, data from 20 drivers are acquired. The results of Hypo-Driver compared to state-of-the-art methods are presented. Compared to the state-of-the-art Hypo-V system, on average, the Hypo-Driver system achieved a detection accuracy (AC) of 96.5%. The obtained results indicate that the Hypo-Driver system based on multimodal and multiview features outperforms other state-of-the-art driver Hypo-V systems by handling many anomalies.Keywords
Cite This Article
Citations
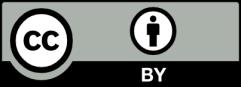