Open Access
ARTICLE
Intelligent Disease Diagnosis Model for Energy Aware Cluster Based IoT Healthcare Systems
1 Department of Information Technology, Faculty of Computing and Information Technology, King Abdulaziz University, Jeddah, Saudi Arabia
2 Department of Computer Science, Faculty of Computing and Information Technology, King Abdulaziz University, Jeddah, Saudi Arabia
3 Department of Mathematics and Statistics, College of Science, Taif University, Taif, 21944, Saudi Arabia
4 Department of Mathematics, Faculty of Science, New Valley University, El-Kharga, 72511, Egypt
* Corresponding Author: Wafaa Alsaggaf. Email:
Computers, Materials & Continua 2022, 71(1), 1189-1203. https://doi.org/10.32604/cmc.2022.022469
Received 08 August 2021; Accepted 09 September 2021; Issue published 03 November 2021
Abstract
In recent days, advancements in the Internet of Things (IoT) and cloud computing (CC) technologies have emerged in different application areas, particularly healthcare. The use of IoT devices in healthcare sector often generates large amount of data and also spent maximum energy for data transmission to the cloud server. Therefore, energy efficient clustering mechanism is needed to effectively reduce the energy consumption of IoT devices. At the same time, the advent of deep learning (DL) models helps to analyze the healthcare data in the cloud server for decision making. With this motivation, this paper presents an intelligent disease diagnosis model for energy aware cluster based IoT healthcare systems, called IDDM-EAC technique. The proposed IDDM-EAC technique involves a 3-stage process namely data acquisition, clustering, and disease diagnosis. In addition, the IDDM-EAC technique derives a chicken swarm optimization based energy aware clustering (CSOEAC) technique to group the IoT devices into clusters and select cluster heads (CHs). Moreover, a new coyote optimization algorithm (COA) with deep belief network (DBN), called COA-DBN technique is employed for the disease diagnostic process. The COA-DBN technique involves the design of hyperparameter optimizer using COA to optimally adjust the parameters involved in the DBN model. In order to inspect the betterment of the IDDM-EAC technique, a wide range of experiments were carried out using real time data from IoT devices and benchmark data from UCI repository. The experimental results demonstrate the promising performance with the minimal total energy consumption of 63% whereas the EEPSOC, ABC, GWO, and ACO algorithms have showcased a higher total energy consumption of 69%, 78%, 83%, and 84% correspondingly.Keywords
Cite This Article
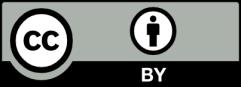