Open Access
Fed-DFE: A Decentralized Function Encryption-Based Privacy-Preserving Scheme for Federated Learning
1 Cyberspace Institute of Advanced Technology (CIAT), Guangzhou University, Guangzhou, China
2 School of Electrical and Data Engineering, University of Technology Sydney, Sydney, Australia
3 Zhejiang Lab, Hangzhou, China
* Corresponding Author: Lihua Yin. Email:
Computers, Materials & Continua 2022, 71(1), 1867-1886. https://doi.org/10.32604/cmc.2022.022290
Received 02 August 2021; Accepted 03 September 2021; Issue published 03 November 2021
Abstract
Federated learning is a distributed learning framework which trains global models by passing model parameters instead of raw data. However, the training mechanism for passing model parameters is still threatened by gradient inversion, inference attacks, etc. With a lightweight encryption overhead, function encryption is a viable secure aggregation technique in federation learning, which is often used in combination with differential privacy. The function encryption in federal learning still has the following problems: a) Traditional function encryption usually requires a trust third party (TTP) to assign the keys. If a TTP colludes with a server, the security aggregation mechanism can be compromised. b) When using differential privacy in combination with function encryption, the evaluation metrics of incentive mechanisms in the traditional federal learning become invisible. In this paper, we propose a hybrid privacy-preserving scheme for federated learning, called Fed-DFE. Specifically, we present a decentralized multi-client function encryption algorithm. It replaces the TTP in traditional function encryption with an interactive key generation algorithm, avoiding the problem of collusion. Then, an embedded incentive mechanism is designed for function encryption. It models the real parameters in federated learning and finds a balance between privacy preservation and model accuracy. Subsequently, we implemented a prototype of Fed-DFE and evaluated the performance of decentralized function encryption algorithm. The experimental results demonstrate the effectiveness and efficiency of our scheme.Keywords
Cite This Article
Citations
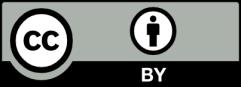
This work is licensed under a Creative Commons Attribution 4.0 International License , which permits unrestricted use, distribution, and reproduction in any medium, provided the original work is properly cited.