Open Access
ARTICLE
Attribute Weighted Naïve Bayes Classifier
Multimedia University, Cyberjaya, 63100, Malaysia
* Corresponding Author: Lee-Kien Foo. Email:
(This article belongs to the Special Issue: Innovations in Artificial Intelligence using Data Mining and Big Data)
Computers, Materials & Continua 2022, 71(1), 1945-1957. https://doi.org/10.32604/cmc.2022.022011
Received 24 July 2021; Accepted 13 September 2021; Issue published 03 November 2021
Abstract
The naïve Bayes classifier is one of the commonly used data mining methods for classification. Despite its simplicity, naïve Bayes is effective and computationally efficient. Although the strong attribute independence assumption in the naïve Bayes classifier makes it a tractable method for learning, this assumption may not hold in real-world applications. Many enhancements to the basic algorithm have been proposed in order to alleviate the violation of attribute independence assumption. While these methods improve the classification performance, they do not necessarily retain the mathematical structure of the naïve Bayes model and some at the expense of computational time. One approach to reduce the naïveté of the classifier is to incorporate attribute weights in the conditional probability. In this paper, we proposed a method to incorporate attribute weights to naïve Bayes. To evaluate the performance of our method, we used the public benchmark datasets. We compared our method with the standard naïve Bayes and baseline attribute weighting methods. Experimental results show that our method to incorporate attribute weights improves the classification performance compared to both standard naïve Bayes and baseline attribute weighting methods in terms of classification accuracy and F1, especially when the independence assumption is strongly violated, which was validated using the Chi-square test of independence.Keywords
Cite This Article
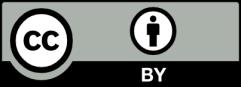
This work is licensed under a Creative Commons Attribution 4.0 International License , which permits unrestricted use, distribution, and reproduction in any medium, provided the original work is properly cited.