Open Access
ARTICLE
Intelligent Biomedical Electrocardiogram Signal Processing for Cardiovascular Disease Diagnosis
1 Department of Electronics and Communication Engineering, University College of Engineering Ariyalur, Ariyalur, 621704, India
2 School of Computing, SRM Institute of Science and Technology, Chennai, 603203, India
3 Department of Electronics and Communication Engineering, K. Ramakrishnan College of Engineering, Tiruchirappalli, 621112, India
4 Department of Computer Science, Factually of Science & Art at Mahayil, King Khalid University, Saudi Arabia
5 Faculty of Computer and IT, Sana'a University, Yemen
6 Department of Biomedical Engineering, College of Engineering, Princess Nourah Bint Abdulrahman University, Saudi Arabia
7 Department of Computer and Self Development, Preparatory Year Deanship, Prince Sattam Bin Abdulaziz University, Alkharj, Saudi Arabia
* Corresponding Author: R. Krishnaswamy. Email:
Computers, Materials & Continua 2022, 71(1), 255-268. https://doi.org/10.32604/cmc.2022.021995
Received 22 July 2021; Accepted 23 August 2021; Issue published 03 November 2021
Abstract
Automatic biomedical signal recognition is an important process for several disease diagnoses. Particularly, Electrocardiogram (ECG) is commonly used to identify cardiovascular diseases. The professionals can determine the existence of cardiovascular diseases using the morphological patterns of the ECG signals. In order to raise the diagnostic accuracy and reduce the diagnostic time, automated computer aided diagnosis model is necessary. With the advancements of artificial intelligence (AI) techniques, large quantity of biomedical datasets can be easily examined for decision making. In this aspect, this paper presents an intelligent biomedical ECG signal processing (IBECG-SP) technique for CVD diagnosis. The proposed IBECG-SP technique examines the ECG signals for decision making. In addition, gated recurrent unit (GRU) model is used for the feature extraction of the ECG signals. Moreover, earthworm optimization (EWO) algorithm is utilized to optimally tune the hyperparameters of the GRU model. Lastly, softmax classifier is employed to allot appropriate class labels to the applied ECG signals. For examining the enhanced outcomes of the proposed IBECG-SP technique, an extensive simulation analysis take place on the PTB-XL database. The experimental results portrayed the supremacy of the IBECG-SP technique over the recent state of art techniques.Keywords
Cite This Article
Citations
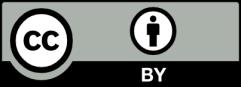