Open Access
ARTICLE
SMOTEDNN: A Novel Model for Air Pollution Forecasting and AQI Classification
1 Department of Computer Science, College of Computer and Information Sciences, Majmaah University Almajmaah, 11952, Saudi Arabia
* Corresponding Author: Mohd Anul Haq. Email:
(This article belongs to the Special Issue: Artificial Intelligence and Machine Learning Algorithms in Real-World Applications and Theories)
Computers, Materials & Continua 2022, 71(1), 1403-1425. https://doi.org/10.32604/cmc.2022.021968
Received 22 July 2021; Accepted 23 August 2021; Issue published 03 November 2021
Abstract
Rapid industrialization and urbanization are rapidly deteriorating ambient air quality, especially in the developing nations. Air pollutants impose a high risk on human health and degrade the environment as well. Earlier studies have used machine learning (ML) and statistical modeling to classify and forecast air pollution. However, these methods suffer from the complexity of air pollution dataset resulting in a lack of efficient classification and forecasting of air pollution. ML-based models suffer from improper data pre-processing, class imbalance issues, data splitting, and hyperparameter tuning. There is a gap in the existing ML-based studies on air pollution due to improper data handling and optimization. The present investigation aims to bridge these gaps and aid in effective air pollution classification and forecasting. Five ML models were developed, including one novel model named SMOTEDNN (Synthetic Minority Oversampling Technique with Deep Neural Network) to address air pollution classification. All five models utilized efficient data pre-processing and rigorous hyperparameter optimization. Three forecasting models were developed to forecast air pollution for one step-index based on statistical autoregression. All developed models in present investigation showed higher accuracy. Significantly, the novel model SMOTEDNN achieved an accuracy of (99.90%) higher than the other models from the current investigation and previous studies.Keywords
Cite This Article
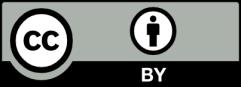
This work is licensed under a Creative Commons Attribution 4.0 International License , which permits unrestricted use, distribution, and reproduction in any medium, provided the original work is properly cited.