Open Access
ARTICLE
ILipo-PseAAC: Identification of Lipoylation Sites Using Statistical Moments and General PseAAC
1 School of Science and Technology, University of Management and Technology, Lahore, Pakistan
2 Department of Computer Science and Information Technology, Virtual University of Pakistan, Lahore, Pakistan
3 Department of Biomedical Engineering, New Jersey Institute of Technology, Newark, NJ, USA
4 Department of Computer Sciences, College of Computer and Information Sciences, Princess Nourah bint Abdulrahman University (PNU), Riyadh, Saudi Arabia
5 Department of Economics, University of Lahore, Lahore, Pakistan
* Corresponding Author: Talha Imtiaz Baig. Email:
(This article belongs to the Special Issue: Machine Learning Applications in Medical, Finance, Education and Cyber Security)
Computers, Materials & Continua 2022, 71(1), 215-230. https://doi.org/10.32604/cmc.2022.021849
Received 17 July 2021; Accepted 18 August 2021; Issue published 03 November 2021
Abstract
Lysine Lipoylation is a protective and conserved Post Translational Modification (PTM) in proteomics research like prokaryotes and eukaryotes. It is connected with many biological processes and closely linked with many metabolic diseases. To develop a perfect and accurate classification model for identifying lipoylation sites at the protein level, the computational methods and several other factors play a key role in this purpose. Usually, most of the techniques and different traditional experimental models have a very high cost. They are time-consuming; so, it is required to construct a predictor model to extract lysine lipoylation sites. This study proposes a model that could predict lysine lipoylation sites with the help of a classification method known as Artificial Neural Network (ANN). The ANN algorithm deals with the noise problem and imbalance classification in lipoylation sites dataset samples. As the result shows in ten-fold cross-validation, a brilliant performance is achieved through the predictor model with an accuracy of 99.88%, and also achieved 0.9976 as the highest value of MCC. So, the predictor model is a very useful and helpful tool for lipoylation sites prediction. Some of the residues around lysine lipoylation sites play a vital part in prediction, as demonstrated during feature analysis. The wonderful results reported through the evaluation and prediction of this model can provide an informative and relative explanation for lipoylation and its molecular mechanisms.Keywords
Cite This Article
Citations
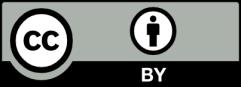
This work is licensed under a Creative Commons Attribution 4.0 International License , which permits unrestricted use, distribution, and reproduction in any medium, provided the original work is properly cited.