Open Access
ARTICLE
A Hybrid Deep Learning Scheme for Multi-Channel Sleep Stage Classification
1 School of Sciences, University of Southern Queensland, Toowoomba, 4350, Australia
2 School of Mechanical and Electrical Engineering, University of Southern Queensland, Toowoomba, 4350, Australia
* Corresponding Author: Siuly Siuly. Email:
(This article belongs to the Special Issue: Advances in Artificial Intelligence and Machine learning in Biomedical and Healthcare Informatics)
Computers, Materials & Continua 2022, 71(1), 889-905. https://doi.org/10.32604/cmc.2022.021830
Received 16 July 2021; Accepted 03 September 2021; Issue published 03 November 2021
Abstract
Sleep stage classification plays a significant role in the accurate diagnosis and treatment of sleep-related diseases. This study aims to develop an efficient deep learning based scheme for correctly identifying sleep stages using multi-biological signals such as electroencephalography (EEG), electrocardiogram (ECG), electromyogram (EMG), and electrooculogram (EOG). Most of the prior studies in sleep stage classification focus on hand-crafted feature extraction methods. Traditional hand-crafted feature extraction methods choose features manually from raw data, which is tedious, and these features are limited in their ability to balance efficiency and accuracy. Moreover, most of the existing works on sleep staging are either single channel (a single-lead EEG may not contain enough information) or only EEG signal based which can not reveal more complicated physical features for reliable classification of various sleep stages. This study proposes an approach to combine Convolutional Neural Networks (CNNs) and Gated Recurrent Units (GRUs) that can discover hidden features from multi-biological signal data to recognize the different sleep stages efficiently. In the proposed scheme, the CNN is designed to extract concealed features from the multi-biological signals, and the GRU is employed to automatically learn the transition rules among different sleep stages. After that, the softmax layers are used to classify various sleep stages. The proposed method was tested on two publicly available databases: Sleep Heart Health Study (SHHS) and St. Vincent's University Hospital/University College Dublin Sleep Apnoea (UCDDB). The experimental results reveal that the proposed model yields better performance compared to state-of-the-art works. Our proposed scheme will assist in building a new system to deal with multi-channel or multi-modal signal processing tasks in various applications.Keywords
Cite This Article
Citations
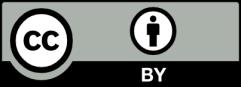
This work is licensed under a Creative Commons Attribution 4.0 International License , which permits unrestricted use, distribution, and reproduction in any medium, provided the original work is properly cited.