Open Access
ARTICLE
Clustering Indoor Location Data for Social Distancing and Human Mobility to Combat COVID-19
1 School of Information Technology, Monash University Malaysia, Jalan Lagoon Selatan, Bandar Sunway, 47500, Selangor Darul Ehsan, Malaysia
2 Faculty of Engineering, Multimedia University, Persiaran Multimedia, Cyberjaya, 63100, Selangor Darul Ehsan, Malaysia
* Corresponding Author: Chee Keong Tan. Email:
(This article belongs to the Special Issue: Application of Artificial Intelligence, Internet of Things, and Learning Approach for Learning Process in COVID-19/Industrial Revolution 4.0)
Computers, Materials & Continua 2022, 71(1), 907-924. https://doi.org/10.32604/cmc.2022.021756
Received 13 July 2021; Accepted 27 August 2021; Issue published 03 November 2021
Abstract
The world is experiencing the unprecedented time of a pandemic caused by the coronavirus disease (i.e., COVID-19). As a countermeasure, contact tracing and social distancing are essential to prevent the transmission of the virus, which can be achieved using indoor location analytics. Based on the indoor location analytics, the human mobility on a site can be monitored and planned to minimize human’s contact and enforce social distancing to contain the transmission of COVID-19. Given the indoor location data, the clustering can be applied to cluster spatial data, spatio-temporal data and movement behavior features for proximity detection or contact tracing applications. More specifically, we propose the Coherent Moving Cluster (CMC) algorithm for contact tracing, the density-based clustering (DBScan) algorithm for identification of hotspots and the trajectory clustering (TRACLUS) algorithm for clustering indoor trajectories. The feature extraction mechanism is then developed to extract useful and valuable features that can assist the proposed system to construct the network of users based on the similarity of the movement behaviors of the users. The network of users is used to model an optimization problem to manage the human mobility on a site. The objective function is formulated to minimize the probability of contact between the users and the optimization problem is solved using the proposed effective scheduling solution based on OR-Tools. The simulation results show that the proposed indoor location analytics system outperforms the existing clustering methods by about 30% in terms of accuracy of clustering trajectories. By adopting this system for human mobility management, the count of close contacts among the users within a confined area can be reduced by 80% in the scenario where all users are allowed to access the site.Keywords
Cite This Article
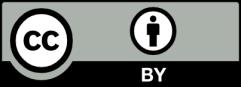
This work is licensed under a Creative Commons Attribution 4.0 International License , which permits unrestricted use, distribution, and reproduction in any medium, provided the original work is properly cited.