Open Access
ARTICLE
Artificial Intelligence Based Sentiment Analysis for Health Crisis Management in Smart Cities
1 Department of Computer and Self Development, Preparatory Year Deanship, Prince Sattam Bin Abdulaziz University, Alkharj, Saudi Arabia
2 Department of Computer Science, College of Computer and Information Sciences, Princess Nourah Bint Abdulrahman University, Saudi Arabia
3 Department of Computer Science, King Khalid University, Muhayel Aseer, Saudi Arabia
4 Faculty of Computer and IT, Sana'a University, Sana'a, Yemen
5 Department of Natural and Applied Sciences, College of Community-Aflaj, Prince Sattam Bin Abdulaziz University, Saudi Arabia
6 School of Computing, Faculty of Engineering, Universiti Teknologi Malaysia, Malaysia
* Corresponding Author: Manar Ahmed Hamza. Email:
Computers, Materials & Continua 2022, 71(1), 143-157. https://doi.org/10.32604/cmc.2022.021502
Received 05 July 2021; Accepted 07 August 2021; Issue published 03 November 2021
Abstract
Smart city promotes the unification of conventional urban infrastructure and information technology (IT) to improve the quality of living and sustainable urban services in the city. To accomplish this, smart cities necessitate collaboration among the public as well as private sectors to install IT platforms to collect and examine massive quantities of data. At the same time, it is essential to design effective artificial intelligence (AI) based tools to handle healthcare crisis situations in smart cities. To offer proficient services to people during healthcare crisis time, the authorities need to look closer towards them. Sentiment analysis (SA) in social networking can provide valuable information regarding public opinion towards government actions. With this motivation, this paper presents a new AI based SA tool for healthcare crisis management (AISA-HCM) in smart cities. The AISA-HCM technique aims to determine the emotions of the people during the healthcare crisis time, such as COVID-19. The proposed AISA-HCM technique involves distinct operations such as pre-processing, feature extraction, and classification. Besides, brain storm optimization (BSO) with deep belief network (DBN), called BSO-DBN model is employed for feature extraction. Moreover, beetle antenna search with extreme learning machine (BAS-ELM) method was utilized for classifying the sentiments as to various classes. The use of BSO and BAS algorithms helps to effectively modify the parameters involved in the DBN and ELM models respectively. The performance validation of the AISA-HCM technique takes place using Twitter data and the outcomes are examined with respect to various measures. The experimental outcomes highlighted the enhanced performance of the AISA-HCM technique over the recent state of art SA approaches with the maximum precision of 0.89, recall of 0.88, F-measure of 0.89, and accuracy of 0.94.Keywords
Cite This Article
Citations
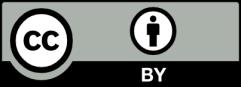