Open Access
ARTICLE
Gaussian Support Vector Machine Algorithm Based Air Pollution Prediction
1 Department of Computer Sceince and Engineering, Karpagam College of Engineering, Coimbatore, 641032, India
2 Department of Electrical and Electronics Engineering, M. Kumarasamy College of Engineering, Karur, 639113, India
3 Department of Applied Cybernetics, Faculty of Science, University of Hradec Králové, Hradec Králové, 50003, Czech Republic
4 Department of Computer Science, College of Computers and Information Technology, Taif University, Taif, 21944, Saudi Arabia
5 Department of Mathematics, Faculty of Science, Mansoura University, Mansoura, 35516, Egypt
6 Department of Computational Mathematics, Science, and Engineering (CMSE), Michigan State University, East Lansing, MI, 48824, USA
7 Department of Electrical and Electronics Engineering, Kongu Engineering College, Erode, 638060, India
* Corresponding Author: Mohamed Abouhawwash. Email:
Computers, Materials & Continua 2022, 71(1), 683-695. https://doi.org/10.32604/cmc.2022.021477
Received 04 July 2021; Accepted 17 August 2021; Issue published 03 November 2021
Abstract
Air pollution is one of the major concerns considering detriments to human health. This type of pollution leads to several health problems for humans, such as asthma, heart issues, skin diseases, bronchitis, lung cancer, and throat and eye infections. Air pollution also poses serious issues to the planet. Pollution from the vehicle industry is the cause of greenhouse effect and CO2 emissions. Thus, real-time monitoring of air pollution in these areas will help local authorities to analyze the current situation of the city and take necessary actions. The monitoring process has become efficient and dynamic with the advancement of the Internet of things and wireless sensor networks. Localization is the main issue in WSNs; if the sensor node location is unknown, then coverage and power and routing are not optimal. This study concentrates on localization-based air pollution prediction systems for real-time monitoring of smart cities. These systems comprise two phases considering the prediction as heavy or light traffic area using the Gaussian support vector machine algorithm based on the air pollutants, such as PM2.5 particulate matter, PM10, nitrogen dioxide (NO2), carbon monoxide (CO), ozone (O3), and sulfur dioxide (SO2). The sensor nodes are localized on the basis of the predicted area using the meta-heuristic algorithms called fast correlation-based elephant herding optimization. The dataset is divided into training and testing parts based on 10 cross-validations. The evaluation on predicting the air pollutant for localization is performed with the training dataset. Mean error prediction in localizing nodes is 9.83 which is lesser than existing solutions and accuracy is 95%.Keywords
Cite This Article
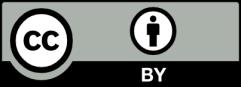
This work is licensed under a Creative Commons Attribution 4.0 International License , which permits unrestricted use, distribution, and reproduction in any medium, provided the original work is properly cited.