Open Access
ARTICLE
Multilingual Sentiment Mining System to Prognosticate Governance
1 COMSATS University Islamabad, Lahore Campus, Lahore, Pakistan
2 Computer Science Department, University of Tabuk, Tabuk, Saudi Arabia
* Corresponding Author: Muhammad Shahid Bhatti. Email:
Computers, Materials & Continua 2022, 71(1), 389-406. https://doi.org/10.32604/cmc.2022.021384
Received 01 July 2021; Accepted 01 August 2021; Issue published 03 November 2021
Abstract
In the age of the internet, social media are connecting us all at the tip of our fingers. People are linkedthrough different social media. The social network, Twitter, allows people to tweet their thoughts on any particular event or a specific political body which provides us with a diverse range of political insights. This paper serves the purpose of text processing of a multilingual dataset including Urdu, English, and Roman Urdu. Explore machine learning solutions for sentiment analysis and train models, collect the data on government from Twitter, apply sentiment analysis, and provide a python library that classifies text sentiment. Training data contained tweets in three languages: English: 200k, Urdu: 200k and Roman Urdu: 11k. Five different classification models are applied to determine sentiments, and eventually, the use of ensemble technique to move forward with the acquired results is explored. The Logistic Regression model performed best with an accuracy of 75%, followed by the Linear Support Vector classifier and Stochastic Gradient Descent model, both having 74% accuracy. Lastly, Multinomial Naïve Bayes and Complement Naïve Bayes models both achieved 73% accuracy.Keywords
Cite This Article
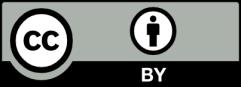
This work is licensed under a Creative Commons Attribution 4.0 International License , which permits unrestricted use, distribution, and reproduction in any medium, provided the original work is properly cited.