Open Access
ARTICLE
Target Detection Algorithm in Crime Recognition Using Artificial Intelligence
Computer Science & Information Technology, Northern Border University, Rafha, Saudi Arabia
* Corresponding Author: Abdulsamad A. AL-Marghilani. Email:
(This article belongs to the Special Issue: Intelligent Big Data Management and Machine Learning Techniques for IoT-Enabled Pervasive Computing)
Computers, Materials & Continua 2022, 71(1), 809-824. https://doi.org/10.32604/cmc.2022.021185
Received 26 June 2021; Accepted 17 August 2021; Issue published 03 November 2021
Abstract
Presently, suspect prediction of crime scenes can be considered as a classification task, which predicts the suspects based on the time, space, and type of crime. Performing digital forensic investigation in a big data environment poses several challenges to the investigational officer. Besides, the facial sketches are widely employed by the law enforcement agencies for assisting the suspect identification of suspects involved in crime scenes. The sketches utilized in the forensic investigations are either drawn by forensic artists or generated through the computer program (composite sketches) based on the verbal explanation given by the eyewitness or victim. Since this suspect identification process is slow and difficult, it is required to design a technique for a quick and automated facial sketch generation. Machine Learning (ML) and deep learning (DL) models find it useful to automatically support the decision of forensics experts. The challenge is the incorporation of the domain expert knowledge with DL models for developing efficient techniques to make better decisions. In this view, this study develops a new artificial intelligence (AI) based DL model with face sketch synthesis (FSS) for suspect identification (DLFSS-SI) in a big data environment. The proposed method performs preprocessing at the primary stage to improvise the image quality. In addition, the proposed model uses a DL based MobileNet (MN) model for feature extractor, and the hyper parameters of the MobileNet are tuned by quasi oppositional firefly optimization (QOFFO) algorithm. The proposed model automatically draws the sketches of the input facial images. Moreover, a qualitative similarity assessment takes place with the sketch drawn by a professional artist by the eyewitness. If there is a higher resemblance between the two sketches, the suspect will be determined. To validate the effective performance of the DLFSS-SI method, a detailed qualitative and quantitative examination takes place. The experimental outcome stated that the DLFSS-SI model has outperformed the compared methods in terms of mean square error (MSE), peak signal to noise ratio (PSNR), average actuary, and average computation time.Keywords
Cite This Article
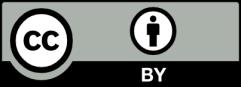