Open Access
ARTICLE
Intelligent Machine Learning Based EEG Signal Classification Model
1 Department of Natural and Applied Sciences, College of Community-Aflaj, Prince Sattam bin Abdulaziz University, Saudi Arabia
2 Department of Information Systems, College of Computer and Information Sciences, Princess Nourah Bint Abdulrahman University, Saudi Arabia
3 Department of Computer Science, King Khalid University, Muhayel Aseer, Saudi Arabia & Faculty of Computer and IT, Sana'a University, Sana'a, Yemen
4 Department of Computer Science, College of Computer, Qassim University, Saudi Arabia
5 Department of Computer and Self Development, Preparatory Year Deanship, Prince Sattam bin Abdulaziz University, Alkharj, Saudi Arabia
* Corresponding Author: Anwer Mustafa Hilal. Email:
Computers, Materials & Continua 2022, 71(1), 1821-1835. https://doi.org/10.32604/cmc.2022.021119
Received 24 June 2021; Accepted 23 August 2021; Issue published 03 November 2021
Abstract
In recent years, Brain-Computer Interface (BCI) system gained much popularity since it aims at establishing the communication between human brain and computer. BCI systems are applied in several research areas such as neuro-rehabilitation, robots, exoeskeletons, etc. Electroencephalography (EEG) is a technique commonly applied in capturing brain signals. It is incorporated in BCI systems since it has attractive features such as non-invasive nature, high time-resolution output, mobility and cost-effective. EEG classification process is highly essential in decision making process and it incorporates different processes namely, feature extraction, feature selection, and classification. With this motivation, the current research paper presents an Intelligent Optimal Fuzzy Support Vector Machine-based EEC recognition (IOFSVM-EEG) model for BCI system. Independent Component Analysis (ICA) technique is applied onto the proposed IOFSVM-EEG model to remove the artefacts that exist in EEG signal and to retain the meaningful EEG information. Besides, Common Spatial Pattern (CSP)-based feature extraction technique is utilized to derive a helpful set of feature vectors from the preprocessed EEG signals. Moreover, OFSVM method is applied in the classification of EEG signals, in which the parameters involved in FSVM are optimally tuned using Grasshopper Optimization Algorithm (GOA). In order to validate the enhanced EEG recognition outcomes of the proposed IOFSVM-EEG model, an extensive set of experiments was conducted. The outcomes were examined under distinct aspects. The experimental results highlighted the enhanced performance of the presented IOFSVM-EEG model over other state-of-the-art methods.Keywords
Cite This Article
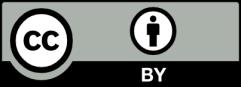
This work is licensed under a Creative Commons Attribution 4.0 International License , which permits unrestricted use, distribution, and reproduction in any medium, provided the original work is properly cited.