Open Access
ARTICLE
Covid-19 CT Lung Image Segmentation Using Adaptive Donkey and Smuggler Optimization Algorithm
1 Department of Computer Science, CHRIST (Deemed to be University), Bengaluru, India
2 Department of Computer Science and Engineering, CHRIST (Deemed to be University), Bengaluru, India
3 Information Systems Department, College of Computer and Information Science, Princess Nourah Bint Abdulrahman University, Riyadh, KSA 84428, Saudi Arabia
4 Department of Mathematics, Faculty of Science, Cairo University, Giza, 12613, Egypt
5 Computer Sciences Department, College of Computer and Information Science, Princess Nourah Bint Abdulrahman University, Riyadh, Saudi Arabia
6 Department of Electrical Engineering, Faculty of Engineering-Shoubra, Benha University, Cairo, Egypt
* Corresponding Author: Ala Saleh Alluhaidan. Email:
Computers, Materials & Continua 2022, 71(1), 1133-1152. https://doi.org/10.32604/cmc.2022.020919
Received 14 June 2021; Accepted 23 August 2021; Issue published 03 November 2021
Abstract
COVID’19 has caused the entire universe to be in existential health crisis by spreading globally in the year 2020. The lungs infection is detected in Computed Tomography (CT) images which provide the best way to increase the existing healthcare schemes in preventing the deadly virus. Nevertheless, separating the infected areas in CT images faces various issues such as low-intensity difference among normal and infectious tissue and high changes in the characteristics of the infection. To resolve these issues, a new inf-Net (Lung Infection Segmentation Deep Network) is designed for detecting the affected areas from the CT images automatically. For the worst segmentation results, the Edge-Attention Representation (EAR) is optimized using Adaptive Donkey and Smuggler Optimization (ADSO). The edges which are identified by the ADSO approach is utilized for calculating dissimilarities. An IFCM (Intuitionistic Fuzzy C-Means) clustering approach is applied for computing the similarity of the EA component among the generated edge maps and Ground-Truth (GT) edge maps. Also, a Semi-Supervised Segmentation (SSS) structure is designed using the Randomly Selected Propagation (RP) technique and Inf-Net, which needs only less number of images and unlabelled data. Semi-Supervised Multi-Class Segmentation (SSMCS) is designed using a Bi-LSTM (Bi-Directional Long-Short-Term-memory), acquires all the advantages of the disease segmentation done using Semi Inf-Net and enhances the execution of multi-class disease labelling. The newly designed SSMCS approach is compared with existing U-Net++, MCS, and Semi-Inf-Net. factors such as MAE (Mean Absolute Error), Structure measure, Specificity (Spec), Dice Similarity coefficient, Sensitivity (Sen), and Enhance-Alignment Measure are considered for evaluation purpose.Keywords
Cite This Article
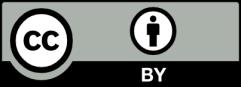
This work is licensed under a Creative Commons Attribution 4.0 International License , which permits unrestricted use, distribution, and reproduction in any medium, provided the original work is properly cited.