Open Access
ARTICLE
Deep Learning-Based Approach for Arabic Visual Speech Recognition
1 Computer Science Department, Faculty of Computing and Information Technology, King Abdulaziz University, Jeddah, 21589, Saudi Arabia
2 Electrical Engineering Department, Faculty of Engineering at Shoubra, Benha University, Cairo, 11629, Egypt
* Corresponding Author: Nadia H. Alsulami. Email:
(This article belongs to the Special Issue: Recent Advances in Deep Learning, Information Fusion, and Features Selection for Video Surveillance Application)
Computers, Materials & Continua 2022, 71(1), 85-108. https://doi.org/10.32604/cmc.2022.019450
Received 14 April 2021; Accepted 03 June 2021; Issue published 03 November 2021
Abstract
Lip-reading technologies are rapidly progressing following the breakthrough of deep learning. It plays a vital role in its many applications, such as: human-machine communication practices or security applications. In this paper, we propose to develop an effective lip-reading recognition model for Arabic visual speech recognition by implementing deep learning algorithms. The Arabic visual datasets that have been collected contains 2400 records of Arabic digits and 960 records of Arabic phrases from 24 native speakers. The primary purpose is to provide a high-performance model in terms of enhancing the preprocessing phase. Firstly, we extract keyframes from our dataset. Secondly, we produce a Concatenated Frame Images (CFIs) that represent the utterance sequence in one single image. Finally, the VGG-19 is employed for visual features extraction in our proposed model. We have examined different keyframes: 10, 15, and 20 for comparing two types of approaches in the proposed model: (1) the VGG-19 base model and (2) VGG-19 base model with batch normalization. The results show that the second approach achieves greater accuracy: 94% for digit recognition, 97% for phrase recognition, and 93% for digits and phrases recognition in the test dataset. Therefore, our proposed model is superior to models based on CFIs input.Keywords
Cite This Article
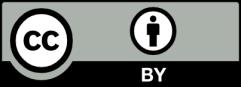